How Simple Data Analysis Can Put Your Data to Work Before You're ML Ready
Join leaders July 26-28 for Transform AI and Edge Week. Hear high-level leaders discuss topics around AL/ML technology, conversational AI, IVA, NLP, Edge, and more. Book your free pass now!
Data has become the new holy grail for business. From fledgling startups to decades-old giants, companies across all industries collect (or hope to collect) large volumes of structured, semi-structured, and unstructured information to improve their core offerings as well as improve operational efficiency. .
The idea that comes right away is the implementation of machine learning, but not every organization immediately has the plan or the resources for mobile data.
"We live in an age where companies are just collecting data, regardless of the use case or what they're going to do with it. And that's exciting, but also a little scary because the volume of data that is collected, and how it is collected, is not necessarily always done with a use case in mind,” Ameen Kazerouni, director of data and analytics at Orangetheory Fitness, said during a session at VentureBeat's Transform 2022 conference.
start smallThe problem is a major obstacle to data-driven growth, but according to Kazerouni, companies don't always have to swim deep and invest heavily in AI and ML from the start. Instead, they can just start small with basic data practices and then scale up.
EventTransform 2022
Sign up now to get your free virtual pass to Transform AI Week, July 26-28. Hear from AI and data leaders at Visa, Lowe's eBay, Credit Karma, Kaiser, Honeywell, Google, Nissan, Toyota, John Deere, and more.
register hereThe executive, who previously led AI efforts at Zappos, said one of the first initiatives in the face of massive volumes of data should be to create a standardized, shared language for discussing the information collected. This is important to ensure that the value derived from the data means the same to every stakeholder.
"I think many CEOs, COOs, and CFOs of companies that have collected large volumes of data run into this problem, where everyone uses the same name for metrics, but the value is different depending on the data source they got it from. And that almost never should be the case,” he noted.
Once the shared language is ready, the next step is to connect with leaders to identify repetitive and time-consuming processes that are handled by domain experts who might otherwise help with more data issues urgent. According to Kazerouni, these processes should be simplified or automated, which will democratize data, making it available to stakeholders for more informed decision-making.
"As this happens, you'll start to see the benefits of your data immediately (and look at the bigger issues), without having to make big technology investments upfront or go, hey , let's find something we can flip machine learning on and work backwards from that,” the exec said.
Centralized star approachFor best results, Kazerouni pointed out that start-ups that aren't tech-native should focus on a hub-and-spoke approach instead of trying to build everything in-house. They just need to focus on one differentiator and use market solutions to get the technology needed to get the job done.
"However, I also think it's important to take the data from that provider and move it internally to a central hub or data lake, which actually uses the data at the point of generation for the purpose of which [they] were generated. for. And if you need to leverage that data elsewhere or connect it to another data asset, bring it to the center...

Join leaders July 26-28 for Transform AI and Edge Week. Hear high-level leaders discuss topics around AL/ML technology, conversational AI, IVA, NLP, Edge, and more. Book your free pass now!
Data has become the new holy grail for business. From fledgling startups to decades-old giants, companies across all industries collect (or hope to collect) large volumes of structured, semi-structured, and unstructured information to improve their core offerings as well as improve operational efficiency. .
The idea that comes right away is the implementation of machine learning, but not every organization immediately has the plan or the resources for mobile data.
"We live in an age where companies are just collecting data, regardless of the use case or what they're going to do with it. And that's exciting, but also a little scary because the volume of data that is collected, and how it is collected, is not necessarily always done with a use case in mind,” Ameen Kazerouni, director of data and analytics at Orangetheory Fitness, said during a session at VentureBeat's Transform 2022 conference.
start smallThe problem is a major obstacle to data-driven growth, but according to Kazerouni, companies don't always have to swim deep and invest heavily in AI and ML from the start. Instead, they can just start small with basic data practices and then scale up.
EventTransform 2022
Sign up now to get your free virtual pass to Transform AI Week, July 26-28. Hear from AI and data leaders at Visa, Lowe's eBay, Credit Karma, Kaiser, Honeywell, Google, Nissan, Toyota, John Deere, and more.
register hereThe executive, who previously led AI efforts at Zappos, said one of the first initiatives in the face of massive volumes of data should be to create a standardized, shared language for discussing the information collected. This is important to ensure that the value derived from the data means the same to every stakeholder.
"I think many CEOs, COOs, and CFOs of companies that have collected large volumes of data run into this problem, where everyone uses the same name for metrics, but the value is different depending on the data source they got it from. And that almost never should be the case,” he noted.
Once the shared language is ready, the next step is to connect with leaders to identify repetitive and time-consuming processes that are handled by domain experts who might otherwise help with more data issues urgent. According to Kazerouni, these processes should be simplified or automated, which will democratize data, making it available to stakeholders for more informed decision-making.
"As this happens, you'll start to see the benefits of your data immediately (and look at the bigger issues), without having to make big technology investments upfront or go, hey , let's find something we can flip machine learning on and work backwards from that,” the exec said.
Centralized star approachFor best results, Kazerouni pointed out that start-ups that aren't tech-native should focus on a hub-and-spoke approach instead of trying to build everything in-house. They just need to focus on one differentiator and use market solutions to get the technology needed to get the job done.
"However, I also think it's important to take the data from that provider and move it internally to a central hub or data lake, which actually uses the data at the point of generation for the purpose of which [they] were generated. for. And if you need to leverage that data elsewhere or connect it to another data asset, bring it to the center...
What's Your Reaction?
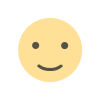
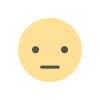
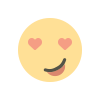
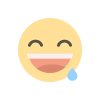
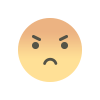
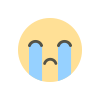
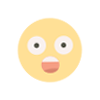