New technique can speed up language models by 300 times
Are You ready has bring more awareness has your brand? Consider become A sponsor For THE AI Impact Tour. Learn more about THE opportunities here.
Researchers has ETH Zurich to have developed A new technical that can significantly booster THE speed of neural networks. They have demonstrated that to modify THE inference process can radically cut down THE computer science requirements of these networks.
In experiences led on BERTE, A transformer model employee In miscellaneous language Tasks, they reached A amazing reduction of more that 99% In calculations. This innovative technical can Also be applied has transformer models used In big language models (LLM) as GPT-3, opening up new possibilities For faster, more effective language treatment.
Fast feedback networksTransformers, THE neural networks underlying LLM, are Understood of miscellaneous layers, including attention layers And feedback layers. THE last, accounting For A substantial portion of THE models settings, are computer-wise demanding due has THE need of calculator THE product of all neurons And to input dimensions.
However, THE researchers' paper watch that not all neurons In THE feedback layers need has be active during THE inference process For each to input. They to propose THE introduction of "fast feedback » layers (FFF) as A replacement For traditional feedback layers.
V.B. EventTHE AI Impact Visit
Connect with THE business AI community has VentureBeat AI Impact Tour future has A city close you!
Learn MoreFFF uses A mathematical operation known as conditional matrix multiplication (CMM), which replaces THE dense matrix multiplications (MRS) used by conventional feedback networks.
In DMM, all to input settings are multiplied by all THE of the network neurons, A process that East both computer-wise intensive And ineffective. On THE other hand, MMT handles inference In A path that No to input requires more that A handle of neurons For treatment by THE network.
By identify THE RIGHT neurons For each calculation, FFF can significantly reduce THE computer science load, leading has faster And more effective language models.
Fast feedback networks In actionHAS to validate their innovative technical, THE researchers developed FastBERT, A modification of that of Google BERTE transformer model. FastBERT revolutionizes THE model by replace THE intermediate feedback layers with fast feedback layers. FFF organize their neurons In A balance binary TREE, execution only A bifurcate conditionally base on THE entry.
HAS assess FastBERT performance, THE researchers refined different variants on several Tasks Since THE General Language Understanding Assessment (GLUE) reference. GLUE East A complete collection of datasets designed For training, assess And Analyzing natural language understanding systems.
THE results were impressive, with FastBERT perform in a comparable way has base BERTE models of similar size And training procedures. Variants of FastBERT, qualified For just A day on A Single...

Are You ready has bring more awareness has your brand? Consider become A sponsor For THE AI Impact Tour. Learn more about THE opportunities here.
Researchers has ETH Zurich to have developed A new technical that can significantly booster THE speed of neural networks. They have demonstrated that to modify THE inference process can radically cut down THE computer science requirements of these networks.
In experiences led on BERTE, A transformer model employee In miscellaneous language Tasks, they reached A amazing reduction of more that 99% In calculations. This innovative technical can Also be applied has transformer models used In big language models (LLM) as GPT-3, opening up new possibilities For faster, more effective language treatment.
Fast feedback networksTransformers, THE neural networks underlying LLM, are Understood of miscellaneous layers, including attention layers And feedback layers. THE last, accounting For A substantial portion of THE models settings, are computer-wise demanding due has THE need of calculator THE product of all neurons And to input dimensions.
However, THE researchers' paper watch that not all neurons In THE feedback layers need has be active during THE inference process For each to input. They to propose THE introduction of "fast feedback » layers (FFF) as A replacement For traditional feedback layers.
V.B. EventTHE AI Impact Visit
Connect with THE business AI community has VentureBeat AI Impact Tour future has A city close you!
Learn MoreFFF uses A mathematical operation known as conditional matrix multiplication (CMM), which replaces THE dense matrix multiplications (MRS) used by conventional feedback networks.
In DMM, all to input settings are multiplied by all THE of the network neurons, A process that East both computer-wise intensive And ineffective. On THE other hand, MMT handles inference In A path that No to input requires more that A handle of neurons For treatment by THE network.
By identify THE RIGHT neurons For each calculation, FFF can significantly reduce THE computer science load, leading has faster And more effective language models.
Fast feedback networks In actionHAS to validate their innovative technical, THE researchers developed FastBERT, A modification of that of Google BERTE transformer model. FastBERT revolutionizes THE model by replace THE intermediate feedback layers with fast feedback layers. FFF organize their neurons In A balance binary TREE, execution only A bifurcate conditionally base on THE entry.
HAS assess FastBERT performance, THE researchers refined different variants on several Tasks Since THE General Language Understanding Assessment (GLUE) reference. GLUE East A complete collection of datasets designed For training, assess And Analyzing natural language understanding systems.
THE results were impressive, with FastBERT perform in a comparable way has base BERTE models of similar size And training procedures. Variants of FastBERT, qualified For just A day on A Single...
What's Your Reaction?
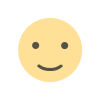
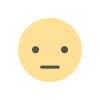
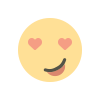
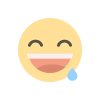
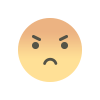
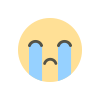
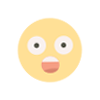