We need to create a better bias in AI
Check out all the Smart Security Summit on-demand sessions here.
At best, AI systems extend and augment the work we do, helping us achieve our goals. At worst, they undermine them. We've all heard of high-profile cases of AI bias, like Amazon's machine learning (ML) recruiting engine discriminating against women or Google Vision's racist results. These cases don't just harm individuals; they go against the original intentions of their creators. Rightly, these examples have caused public outcry and, as a result, have turned the perception of AI bias into something categorically wrong and one that we need to eliminate.
While most people agree on the need to create reliable and fair AI systems, it is unrealistic to eliminate all biases from AI. In fact, as the new wave of ML models move beyond determinism, they are actively designed with some level of built-in subjectivity. Today's most sophisticated systems synthesize input, contextualize content, and interpret results. Rather than trying to completely eliminate bias, organizations should seek to better understand and measure subjectivity.
In support of subjectivityAs ML systems become more sophisticated and our goals become more ambitious, organizations openly demand that they be subjective, albeit in a way that aligns with the overall intent and goals of the project .
We see this clearly in the area of conversational AI, for example. Text-to-speech systems capable of transcribing a video or a call are now common. By comparison, the emerging wave of solutions not only report the discourse, but also interpret and summarize it. So, rather than just transcription, these systems work alongside humans to extend the way they already work, for example, by summarizing a meeting and then creating a list of actions that flow from it.
EventOn-Demand Smart Security Summit
Learn about the essential role of AI and ML in cybersecurity and industry-specific case studies. Watch the on-demand sessions today.
look hereIn these examples, as in many other AI use cases, the system must understand the context and interpret what is important and what can be ignored. In other words, we're building AI systems to act like humans, and subjectivity is part of the whole thing.
The business of biasEven the technological leap that took us from text-to-speech to conversational intelligence in just a few years pales in comparison to the future potential of this branch of AI.
Consider this: Meaning in conversation is, for the most part, conveyed through non-verbal cues and tone, according to Professor Albert Mehrabian in his landmark work, Silent Messages< /em >. Less than ten percent is due to the words themselves. Yet the vast majority of conversational intelligence solutions rely heavily on text interpretation, largely ignoring (for now) contextual cues.
As these intelligence systems begin to interpret what we might call the metadata of human conversation. That is, tone, pauses, context, facial expressions, etc., bias - or intentional, guided subjectivity - is not just a requirement, it is the value proposition.
Conversational intelligence is just one of many areas of machine learning. Some of the most interesting and potentially profitable applications of AI are not to faithfully reproduce what already exists, but rather to interpret...

Check out all the Smart Security Summit on-demand sessions here.
At best, AI systems extend and augment the work we do, helping us achieve our goals. At worst, they undermine them. We've all heard of high-profile cases of AI bias, like Amazon's machine learning (ML) recruiting engine discriminating against women or Google Vision's racist results. These cases don't just harm individuals; they go against the original intentions of their creators. Rightly, these examples have caused public outcry and, as a result, have turned the perception of AI bias into something categorically wrong and one that we need to eliminate.
While most people agree on the need to create reliable and fair AI systems, it is unrealistic to eliminate all biases from AI. In fact, as the new wave of ML models move beyond determinism, they are actively designed with some level of built-in subjectivity. Today's most sophisticated systems synthesize input, contextualize content, and interpret results. Rather than trying to completely eliminate bias, organizations should seek to better understand and measure subjectivity.
In support of subjectivityAs ML systems become more sophisticated and our goals become more ambitious, organizations openly demand that they be subjective, albeit in a way that aligns with the overall intent and goals of the project .
We see this clearly in the area of conversational AI, for example. Text-to-speech systems capable of transcribing a video or a call are now common. By comparison, the emerging wave of solutions not only report the discourse, but also interpret and summarize it. So, rather than just transcription, these systems work alongside humans to extend the way they already work, for example, by summarizing a meeting and then creating a list of actions that flow from it.
EventOn-Demand Smart Security Summit
Learn about the essential role of AI and ML in cybersecurity and industry-specific case studies. Watch the on-demand sessions today.
look hereIn these examples, as in many other AI use cases, the system must understand the context and interpret what is important and what can be ignored. In other words, we're building AI systems to act like humans, and subjectivity is part of the whole thing.
The business of biasEven the technological leap that took us from text-to-speech to conversational intelligence in just a few years pales in comparison to the future potential of this branch of AI.
Consider this: Meaning in conversation is, for the most part, conveyed through non-verbal cues and tone, according to Professor Albert Mehrabian in his landmark work, Silent Messages< /em >. Less than ten percent is due to the words themselves. Yet the vast majority of conversational intelligence solutions rely heavily on text interpretation, largely ignoring (for now) contextual cues.
As these intelligence systems begin to interpret what we might call the metadata of human conversation. That is, tone, pauses, context, facial expressions, etc., bias - or intentional, guided subjectivity - is not just a requirement, it is the value proposition.
Conversational intelligence is just one of many areas of machine learning. Some of the most interesting and potentially profitable applications of AI are not to faithfully reproduce what already exists, but rather to interpret...
What's Your Reaction?
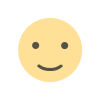
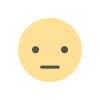
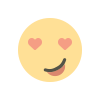
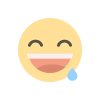
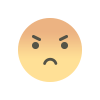
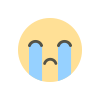
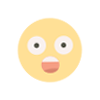