Chaos Seekers Can Now Predict Perilous Tipping Points
Predicting complex systems like the weather is notoriously difficult. But at least the equations that govern the weather don't change from day to day. In contrast, some complex systems can experience "tipping point" transitions, suddenly changing their behavior dramatically and possibly irreversibly, with little warning and potentially catastrophic consequences.
On long enough time scales, most real-world systems are like this. Consider the Gulf Stream in the North Atlantic, which carries warm equatorial water north as part of an ocean conveyor belt that helps regulate Earth's climate. The equations that describe these circulating currents are slowly changing due to the influx of fresh water from the melting ice caps. So far the traffic has gradually slowed down, but in decades it could come to an abrupt halt.
"Let's assume everything is fine now," said Ying-Cheng Lai, a physicist at Arizona State University. "How can you say it's not going to be okay in the future?"
In a series of recent papers, researchers have shown that machine learning algorithms can predict tipping point transitions in archetypal examples of these "non-stationary" systems, as well as the characteristics of their behavior after that they flipped. These surprisingly powerful new techniques could one day find applications in climate science, ecology, epidemiology and many other fields.
Renewed interest in the problem began four years ago with groundbreaking results from the group of Edward Ott, a leading chaos researcher at the University of Maryland. Ott's team found that a type of machine learning algorithm called a recurrent neural network could predict the evolution of stationary chaotic systems (which don't have tipping points) in the surprisingly distant future. The network relied solely on records of the chaotic system's past behavior - it had no information about the underlying equations.
The network's approach to learning differs from that of deep neural networks, which pass data through a large stack of layers of artificial neurons for tasks such as speech recognition and natural language processing. All neural networks learn by adjusting the strength of the connections between their neurons in response to training data. Ott and his collaborators used a less computationally expensive training method called reservoir computation, which adjusts only a few connections in a single layer of artificial neurons. Despite its simplicity, reservoir computing seems suited to the task of predicting chaotic evolution.
As impressive as the 2018 results were, the researchers suspected that the machine learning data-driven approach would not be able to predict tipping point transitions in non-stationary systems or infer how these systems would behave afterwards. A neural network trains on past data about an evolving system, but "what happens in the future evolves by different rules," Ott said. It's like trying to predict the outcome of a baseball game only to find it turns into a cricket game.
And yet, over the past two years, Ott's group and several others have shown that reservoir computing also works in unexpected ways for these systems.
In a 2021 paper, Lai and coworkers gave their reservoir calculation algorithm access to the slowly drifting value of a parameter that would eventually send a model system above a point of failover, but they did not provide any further information on the governing equations of the system. This situation relates to a number of real-life scenarios: we know how the concentration of carbon dioxide in the atmosphere is increasing, for example, but we don't know all the ways this variable will influence the climate. The team found that a neural network trained on past data could predict the value at which the system would eventually become unstable. Ott's group published related results last year.
In a new paper, posted online in July and currently undergoing peer review, Ott and his graduate student Dhruvit Patel explored the predictive power of neural networks that only see the behavior of a system and don't know anything about the underlying parameter responsible for driving a switch-point transition. They fed their recorded neural network data into a simulated system as the hidden parameter drifted, unbeknownst to the network. Remarkably, in many cases the algorithm could both predict...

Predicting complex systems like the weather is notoriously difficult. But at least the equations that govern the weather don't change from day to day. In contrast, some complex systems can experience "tipping point" transitions, suddenly changing their behavior dramatically and possibly irreversibly, with little warning and potentially catastrophic consequences.
On long enough time scales, most real-world systems are like this. Consider the Gulf Stream in the North Atlantic, which carries warm equatorial water north as part of an ocean conveyor belt that helps regulate Earth's climate. The equations that describe these circulating currents are slowly changing due to the influx of fresh water from the melting ice caps. So far the traffic has gradually slowed down, but in decades it could come to an abrupt halt.
"Let's assume everything is fine now," said Ying-Cheng Lai, a physicist at Arizona State University. "How can you say it's not going to be okay in the future?"
In a series of recent papers, researchers have shown that machine learning algorithms can predict tipping point transitions in archetypal examples of these "non-stationary" systems, as well as the characteristics of their behavior after that they flipped. These surprisingly powerful new techniques could one day find applications in climate science, ecology, epidemiology and many other fields.
Renewed interest in the problem began four years ago with groundbreaking results from the group of Edward Ott, a leading chaos researcher at the University of Maryland. Ott's team found that a type of machine learning algorithm called a recurrent neural network could predict the evolution of stationary chaotic systems (which don't have tipping points) in the surprisingly distant future. The network relied solely on records of the chaotic system's past behavior - it had no information about the underlying equations.
The network's approach to learning differs from that of deep neural networks, which pass data through a large stack of layers of artificial neurons for tasks such as speech recognition and natural language processing. All neural networks learn by adjusting the strength of the connections between their neurons in response to training data. Ott and his collaborators used a less computationally expensive training method called reservoir computation, which adjusts only a few connections in a single layer of artificial neurons. Despite its simplicity, reservoir computing seems suited to the task of predicting chaotic evolution.
As impressive as the 2018 results were, the researchers suspected that the machine learning data-driven approach would not be able to predict tipping point transitions in non-stationary systems or infer how these systems would behave afterwards. A neural network trains on past data about an evolving system, but "what happens in the future evolves by different rules," Ott said. It's like trying to predict the outcome of a baseball game only to find it turns into a cricket game.
And yet, over the past two years, Ott's group and several others have shown that reservoir computing also works in unexpected ways for these systems.
In a 2021 paper, Lai and coworkers gave their reservoir calculation algorithm access to the slowly drifting value of a parameter that would eventually send a model system above a point of failover, but they did not provide any further information on the governing equations of the system. This situation relates to a number of real-life scenarios: we know how the concentration of carbon dioxide in the atmosphere is increasing, for example, but we don't know all the ways this variable will influence the climate. The team found that a neural network trained on past data could predict the value at which the system would eventually become unstable. Ott's group published related results last year.
In a new paper, posted online in July and currently undergoing peer review, Ott and his graduate student Dhruvit Patel explored the predictive power of neural networks that only see the behavior of a system and don't know anything about the underlying parameter responsible for driving a switch-point transition. They fed their recorded neural network data into a simulated system as the hidden parameter drifted, unbeknownst to the network. Remarkably, in many cases the algorithm could both predict...
What's Your Reaction?
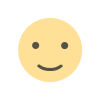
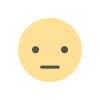
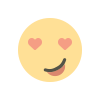
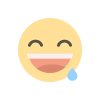
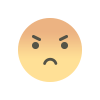
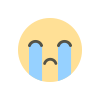
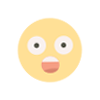