How to choose the right NLP solution
Couldn't attend Transform 2022? Check out all the summit sessions in our on-demand library now! Look here.
For decades, companies have used jury-designed software for structured data when trying to solve problems with unstructured textual data. Although these solutions worked poorly, there was nothing else. Recently, however, machine learning (ML) has improved dramatically to understand natural language.
Unsurprisingly, Silicon Valley is in a frantic race to create market-leading offerings for this new opportunity. Khosla Ventures believes that natural language processing (NLP) is the most important technology trend of the next five years. If the 2000s was about becoming a big data-based business and the 2010s was about becoming a data science-based business, then the 2020s is about becoming a natural language-based business.
To accelerate its transformation into such a business, an organization must establish a viable strategy that aligns with its business goals and drives business impact. Although this may seem like a complex decision that requires an expensive management consulting firm, it is not. It starts with how you answer two questions: First, who employs data scientists and machine learning (MLE) engineers? Second, who builds and operates the underlying ML stack that hosts the relevant models and tools?
A "build it yourself" strategy allows companies to build custom ML models from their data. It also minimizes security risks because companies don't have to share data with external vendors to label or process it. If you can achieve it and afford it, "building your own" leads to substantial competitive advantages, as you now have a world-class artificial intelligence (AI) team, amplifying the productivity in all aspects of the business.
EventMetaBeat 2022
MetaBeat will bring together thought leaders to advise on how metaverse technology will transform the way all industries communicate and do business on October 4 in San Francisco, CA.
register hereHowever, this strategy is by far the most expensive. Building and operating an ML stack is complicated and requires specialized expertise. KPMG estimates that to develop mature AI capabilities, a company needs to employ at least 500-600 full-time AI employees — a majority of whom build and operate the ML stack — and pay them a cumulative $100-120 million. dollars per year. On top of that, there is no guarantee of success, as producing AI is a challenge even for the best teams.
The "Low-code ML platform and pre-trained models" strategy reduces the cost of developing mature AI capabilities, as the vendor handles the majority of the development and operation of the ML stack . Instead of spending more than $100 million per year, organizations can probably reduce that amount to $25-50 million per year. This strategy also allows companies to create custom ML and NLP models.
Although, like the previous strategy, there is no guarantee of success because it does...

Couldn't attend Transform 2022? Check out all the summit sessions in our on-demand library now! Look here.
For decades, companies have used jury-designed software for structured data when trying to solve problems with unstructured textual data. Although these solutions worked poorly, there was nothing else. Recently, however, machine learning (ML) has improved dramatically to understand natural language.
Unsurprisingly, Silicon Valley is in a frantic race to create market-leading offerings for this new opportunity. Khosla Ventures believes that natural language processing (NLP) is the most important technology trend of the next five years. If the 2000s was about becoming a big data-based business and the 2010s was about becoming a data science-based business, then the 2020s is about becoming a natural language-based business.
To accelerate its transformation into such a business, an organization must establish a viable strategy that aligns with its business goals and drives business impact. Although this may seem like a complex decision that requires an expensive management consulting firm, it is not. It starts with how you answer two questions: First, who employs data scientists and machine learning (MLE) engineers? Second, who builds and operates the underlying ML stack that hosts the relevant models and tools?
A "build it yourself" strategy allows companies to build custom ML models from their data. It also minimizes security risks because companies don't have to share data with external vendors to label or process it. If you can achieve it and afford it, "building your own" leads to substantial competitive advantages, as you now have a world-class artificial intelligence (AI) team, amplifying the productivity in all aspects of the business.
EventMetaBeat 2022
MetaBeat will bring together thought leaders to advise on how metaverse technology will transform the way all industries communicate and do business on October 4 in San Francisco, CA.
register hereHowever, this strategy is by far the most expensive. Building and operating an ML stack is complicated and requires specialized expertise. KPMG estimates that to develop mature AI capabilities, a company needs to employ at least 500-600 full-time AI employees — a majority of whom build and operate the ML stack — and pay them a cumulative $100-120 million. dollars per year. On top of that, there is no guarantee of success, as producing AI is a challenge even for the best teams.
The "Low-code ML platform and pre-trained models" strategy reduces the cost of developing mature AI capabilities, as the vendor handles the majority of the development and operation of the ML stack . Instead of spending more than $100 million per year, organizations can probably reduce that amount to $25-50 million per year. This strategy also allows companies to create custom ML and NLP models.
Although, like the previous strategy, there is no guarantee of success because it does...
What's Your Reaction?
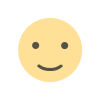
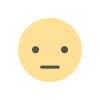
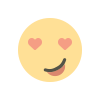
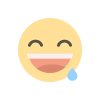
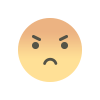
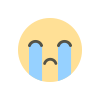
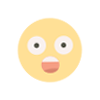