Google's Muse model could be the next big thing for generative AI
Check out all the Smart Security Summit on-demand sessions here.
2022 has been a great year for generative AI, with the release of models such as DALL-E 2, Stable Diffusion, Imagen and Parti. And 2023 seems to be heading that way as Google introduced its latest text-to-image synthesis model, Muse, earlier this month.
Like other text-to-image models, Muse is a deep neural network that takes a text prompt as input and generates an image that matches the description. However, what sets Muse apart from its predecessors is its efficiency and accuracy. By building on the experience of previous work in the field and adding new techniques, Google researchers were able to create a generative model that requires fewer computational resources and makes progress on some of the problems that others suffer from. generative models.
Google's Muse uses token-based image generationMuse builds on previous research in deep learning, including large language models (LLMs), quantized generative networks, and hidden generative image transformers.
"A strong motivation was our interest in unifying the generation of images and text through the use of tokens," said Google researcher Dilip Krishnan. "Muse is built on ideas in MaskGit, a previous post from our group, and masking modeling ideas from large language models."
EventOn-Demand Smart Security Summit
Learn about the essential role of AI and ML in cybersecurity and industry-specific case studies. Watch the on-demand sessions today.
look hereMuse leverages conditioning on pre-trained language models used in previous work, as well as the idea of cascading models, which it borrows from Imagen. One of the interesting differences between Muse and other similar models is the generation of discrete tokens instead of pixel-level representations, which makes the model output much more stable.
Like other text-to-image generators, Muse is trained on a large corpus of image caption pairs. A pre-trained LLM processes the legend and generates an embedded, multi-dimensional digital representation of the textual description. At the same time, a cascade of two image encoder-decoders transforms different resolutions of the input image into a matrix of quantized tokens.
During training, the model trains a base transformer and a super-resolution transformer to align text seamless vector representations with image tokens and use them to render the image. The model adjusts its parameters by randomly hiding image tokens and trying to predict them.
Once trained, the model can generate the image tokens from the text embedding of a new prompt and use image tokens to create new high resolution images.
According to Krishnan, one of Muse's innovations is parallel decoding in symbolic space, which is fundamentally different from diffusion and autoregressive models. Diffusion models use progressive denoising. Autoregressive models use serial decoding. Parallel decoding in Muse provides very good efficiency without loss of visual quality.
"We consider Mu...

Check out all the Smart Security Summit on-demand sessions here.
2022 has been a great year for generative AI, with the release of models such as DALL-E 2, Stable Diffusion, Imagen and Parti. And 2023 seems to be heading that way as Google introduced its latest text-to-image synthesis model, Muse, earlier this month.
Like other text-to-image models, Muse is a deep neural network that takes a text prompt as input and generates an image that matches the description. However, what sets Muse apart from its predecessors is its efficiency and accuracy. By building on the experience of previous work in the field and adding new techniques, Google researchers were able to create a generative model that requires fewer computational resources and makes progress on some of the problems that others suffer from. generative models.
Google's Muse uses token-based image generationMuse builds on previous research in deep learning, including large language models (LLMs), quantized generative networks, and hidden generative image transformers.
"A strong motivation was our interest in unifying the generation of images and text through the use of tokens," said Google researcher Dilip Krishnan. "Muse is built on ideas in MaskGit, a previous post from our group, and masking modeling ideas from large language models."
EventOn-Demand Smart Security Summit
Learn about the essential role of AI and ML in cybersecurity and industry-specific case studies. Watch the on-demand sessions today.
look hereMuse leverages conditioning on pre-trained language models used in previous work, as well as the idea of cascading models, which it borrows from Imagen. One of the interesting differences between Muse and other similar models is the generation of discrete tokens instead of pixel-level representations, which makes the model output much more stable.
Like other text-to-image generators, Muse is trained on a large corpus of image caption pairs. A pre-trained LLM processes the legend and generates an embedded, multi-dimensional digital representation of the textual description. At the same time, a cascade of two image encoder-decoders transforms different resolutions of the input image into a matrix of quantized tokens.
During training, the model trains a base transformer and a super-resolution transformer to align text seamless vector representations with image tokens and use them to render the image. The model adjusts its parameters by randomly hiding image tokens and trying to predict them.
Once trained, the model can generate the image tokens from the text embedding of a new prompt and use image tokens to create new high resolution images.
According to Krishnan, one of Muse's innovations is parallel decoding in symbolic space, which is fundamentally different from diffusion and autoregressive models. Diffusion models use progressive denoising. Autoregressive models use serial decoding. Parallel decoding in Muse provides very good efficiency without loss of visual quality.
"We consider Mu...
What's Your Reaction?
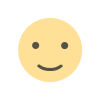
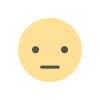
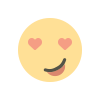
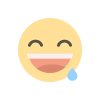
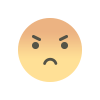
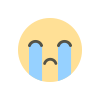
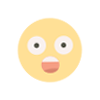