Predictive analytics could be the future, but first we need to solve the data problem
"Your voice is breaking." "We lost you a minute there."
How many times have we all heard or said these things? Or what about the endless buffer white wheel? We've all experienced the endless glitches, crashes, and broken app experiences that affect us more than we care to admit.
For businesses, the move to the cloud and reliance on SaaS applications has made the internet the backbone of business. The internet is the digital supply chain that ensures users have a great digital experience. But there's no certainty as to whether your application and its multitude of components spread across multiple cloud environments actually perform up to scratch.
So for today's organizations looking to be more proactive and automated in how they operate and manage their environments: is delivering a predictable digital experience in an unpredictable internet environment even the cards ? I would say the answer is yes, but only if you solve the data problem first.
The data problem that is the InternetIT operations are overwhelming right now. In today's connected world, where every business, app and device depends on a digital connection every hour of every day, delivering superior digital experiences is essential. But with apps running in the cloud and accessible from many remote endpoints, the number of new blind spots has created huge challenges for anyone tasked with solving user experience issues. This complexity creates a network model plagued by reactive troubleshooting, and the user experience is steadily degraded.
Network professionals tell us that responding to disruptions and meeting new business needs are their top two networking challenges. For these companies, the pursuit of predictive intelligence is about moving from reactive to preventive, thereby identifying problems before they begin to affect the user experience. Predicting and regaining control over what's happening in the cloud is now at the heart of the enterprise network.
Predictive Intelligence: Unlocking Efficiencies and OpportunitiesBut predictive intelligence promises real productivity gains. For organizations with a hybrid workforce, the gains can be significant. Predictively identifying and resolving a single service affecting an outage, such as changing the provider and path that carries application traffic during peak periods, could save a single employee hours of downtime or performance degradation. Multiplied by the employee base, this number quickly becomes significant.
The same goes for satisfying consumer demand. In the age of exponential choice, proactively preventing disruption is key to delivering the always-on digital experience shoppers need and demand. In fact, expectations for digital experiences have skyrocketed.
Unlocking efficiencies and opportunities to increase brand value is the true return on investment of predictive intelligence.
Meeting the Data Challenge for Predictive IntelligenceTroubleshooting is a largely reactive enterprise based on analysis and informed decision-making to improve situations or uncover potential root causes of an active incident.
Determining what is going or went wrong serves an immediate need, but it does nothing to escape that cycle of users abandoning your application late or an unavailable cloud service.
That's the promise of the predictive internet: the ability to leverage a rich set of data and visualizations to analyze historical patterns across a complex mesh of proprietary and third-party networks to predict outages or loss. degradation of services and to take corrective action before the effects manifest themselves. felt by users.
Predictive intelligence at this level is both a data problem and a scale problem. Resolving them is key to making them an achievable reality.
It takes an enormous amount of data to predict the onset of performance degradation or deterioration with a high degree of accuracy. Although the volume of data needed to train a model has been around for a while, the data was often not as clean as it should have been. This caused flow effects in the statistical models. Without good data, the models simply weren't able to produce granular analysis...

"Your voice is breaking." "We lost you a minute there."
How many times have we all heard or said these things? Or what about the endless buffer white wheel? We've all experienced the endless glitches, crashes, and broken app experiences that affect us more than we care to admit.
For businesses, the move to the cloud and reliance on SaaS applications has made the internet the backbone of business. The internet is the digital supply chain that ensures users have a great digital experience. But there's no certainty as to whether your application and its multitude of components spread across multiple cloud environments actually perform up to scratch.
So for today's organizations looking to be more proactive and automated in how they operate and manage their environments: is delivering a predictable digital experience in an unpredictable internet environment even the cards ? I would say the answer is yes, but only if you solve the data problem first.
The data problem that is the InternetIT operations are overwhelming right now. In today's connected world, where every business, app and device depends on a digital connection every hour of every day, delivering superior digital experiences is essential. But with apps running in the cloud and accessible from many remote endpoints, the number of new blind spots has created huge challenges for anyone tasked with solving user experience issues. This complexity creates a network model plagued by reactive troubleshooting, and the user experience is steadily degraded.
Network professionals tell us that responding to disruptions and meeting new business needs are their top two networking challenges. For these companies, the pursuit of predictive intelligence is about moving from reactive to preventive, thereby identifying problems before they begin to affect the user experience. Predicting and regaining control over what's happening in the cloud is now at the heart of the enterprise network.
Predictive Intelligence: Unlocking Efficiencies and OpportunitiesBut predictive intelligence promises real productivity gains. For organizations with a hybrid workforce, the gains can be significant. Predictively identifying and resolving a single service affecting an outage, such as changing the provider and path that carries application traffic during peak periods, could save a single employee hours of downtime or performance degradation. Multiplied by the employee base, this number quickly becomes significant.
The same goes for satisfying consumer demand. In the age of exponential choice, proactively preventing disruption is key to delivering the always-on digital experience shoppers need and demand. In fact, expectations for digital experiences have skyrocketed.
Unlocking efficiencies and opportunities to increase brand value is the true return on investment of predictive intelligence.
Meeting the Data Challenge for Predictive IntelligenceTroubleshooting is a largely reactive enterprise based on analysis and informed decision-making to improve situations or uncover potential root causes of an active incident.
Determining what is going or went wrong serves an immediate need, but it does nothing to escape that cycle of users abandoning your application late or an unavailable cloud service.
That's the promise of the predictive internet: the ability to leverage a rich set of data and visualizations to analyze historical patterns across a complex mesh of proprietary and third-party networks to predict outages or loss. degradation of services and to take corrective action before the effects manifest themselves. felt by users.
Predictive intelligence at this level is both a data problem and a scale problem. Resolving them is key to making them an achievable reality.
It takes an enormous amount of data to predict the onset of performance degradation or deterioration with a high degree of accuracy. Although the volume of data needed to train a model has been around for a while, the data was often not as clean as it should have been. This caused flow effects in the statistical models. Without good data, the models simply weren't able to produce granular analysis...
What's Your Reaction?
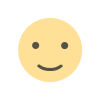
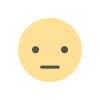
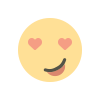
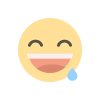
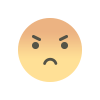
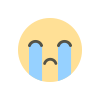
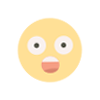