Quantum Machine Learning (QML) Set to Leapfrog in 2023
Check out all the Smart Security Summit on-demand sessions here.
Classical machine learning (ML) algorithms have proven to be powerful tools for a wide range of tasks, including image and speech recognition, natural language processing (NLP), and modeling predictive. However, classical algorithms are limited by the constraints of classical computing and may struggle to process large and complex data sets or achieve high levels of accuracy and precision.
Enter Quantum Machine Learning (QML).
QML combines the power of quantum computing with the predictive capabilities of ML to overcome the limitations of classical algorithms and deliver performance improvements. In their paper "On the role of entanglement in speeding up quantum computing", Richard Jozsa and Neil Linden of the University of Bristol in the UK write that "QML algorithms promise to deliver exponential speedups by compared to their traditional performances". peers for certain tasks, such as data classification, feature selection, and cluster analysis. In particular, the use of quantum algorithms for supervised and unsupervised learning has the potential to revolutionize machine learning and artificial intelligence."
Zohra Ladha, Senior Director, Data Science and AI at Tredence, explains that QML differs from traditional machine learning in several ways:
EventOn-Demand Smart Security Summit
Learn about the essential role of AI and ML in cybersecurity and industry-specific case studies. Watch the on-demand sessions today.
look here Quantum parallelism: Quantum algorithms can take advantage of the unique property of quantum systems known as quantum parallelism, which allows them to perform multiple calculations simultaneously. When processing large amounts of data, such as images or speech, this can dramatically reduce the time it takes to resolve a problem. Quantum superposition: Quantum superposition allows a quantum algorithm to represent multiple states simultaneously. This can allow him to explore possible solutions to a problem, leading to more precise and effective solutions. Quantum entanglement: Quantum algorithms can also use the property of quantum entanglement, which allows quantum systems to be correlated in ways that classical physics cannot explain. This can allow quantum algorithms to perform certain tasks more efficiently than classical algorithms.Traditional machine learning algorithms, which rely on classical computing techniques and lack these quantum capabilities, may be slower or less accurate in some cases.
Research in quantum machine learning began in the 1980s. In the late 1990s and early 2000s, researchers developed quantum neural networks to demonstrate the potential of quantum systems for learning. machine learning that can be trained to recognize patterns in data. These networks have since been applied to a wide range of real-world problems.
A decade later, researchers have developed quantum algorithms and software tools for machine learning tasks. These included quantum versions of popular machine learning algorithms such as support vector machines, decision trees, and neu...

Check out all the Smart Security Summit on-demand sessions here.
Classical machine learning (ML) algorithms have proven to be powerful tools for a wide range of tasks, including image and speech recognition, natural language processing (NLP), and modeling predictive. However, classical algorithms are limited by the constraints of classical computing and may struggle to process large and complex data sets or achieve high levels of accuracy and precision.
Enter Quantum Machine Learning (QML).
QML combines the power of quantum computing with the predictive capabilities of ML to overcome the limitations of classical algorithms and deliver performance improvements. In their paper "On the role of entanglement in speeding up quantum computing", Richard Jozsa and Neil Linden of the University of Bristol in the UK write that "QML algorithms promise to deliver exponential speedups by compared to their traditional performances". peers for certain tasks, such as data classification, feature selection, and cluster analysis. In particular, the use of quantum algorithms for supervised and unsupervised learning has the potential to revolutionize machine learning and artificial intelligence."
Zohra Ladha, Senior Director, Data Science and AI at Tredence, explains that QML differs from traditional machine learning in several ways:
EventOn-Demand Smart Security Summit
Learn about the essential role of AI and ML in cybersecurity and industry-specific case studies. Watch the on-demand sessions today.
look here Quantum parallelism: Quantum algorithms can take advantage of the unique property of quantum systems known as quantum parallelism, which allows them to perform multiple calculations simultaneously. When processing large amounts of data, such as images or speech, this can dramatically reduce the time it takes to resolve a problem. Quantum superposition: Quantum superposition allows a quantum algorithm to represent multiple states simultaneously. This can allow him to explore possible solutions to a problem, leading to more precise and effective solutions. Quantum entanglement: Quantum algorithms can also use the property of quantum entanglement, which allows quantum systems to be correlated in ways that classical physics cannot explain. This can allow quantum algorithms to perform certain tasks more efficiently than classical algorithms.Traditional machine learning algorithms, which rely on classical computing techniques and lack these quantum capabilities, may be slower or less accurate in some cases.
Research in quantum machine learning began in the 1980s. In the late 1990s and early 2000s, researchers developed quantum neural networks to demonstrate the potential of quantum systems for learning. machine learning that can be trained to recognize patterns in data. These networks have since been applied to a wide range of real-world problems.
A decade later, researchers have developed quantum algorithms and software tools for machine learning tasks. These included quantum versions of popular machine learning algorithms such as support vector machines, decision trees, and neu...
What's Your Reaction?
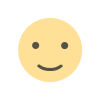
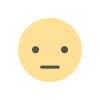
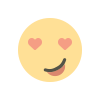
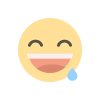
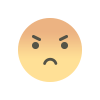
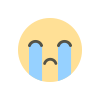
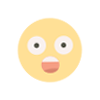