Solving the problem of unstructured data with machine learning
Couldn't attend Transform 2022? Check out all the summit sessions in our on-demand library now! Look here.
We are in the midst of a data revolution. The volume of digital data created over the next five years will total double the amount produced so far – and unstructured data will define this new era of digital experiences.
Unstructured data (information that does not follow conventional patterns or fit into structured database formats) accounts for more than 80% of all new enterprise data. To prepare for this change, companies are finding innovative ways to manage, analyze and maximize the use of data in everything from business analytics to artificial intelligence (AI). But decision makers also face an age-old problem: how to maintain and improve the quality of large, unwieldy datasets?
With machine learning (ML), that's how it is. Advances in ML technology now allow organizations to efficiently process unstructured data and improve quality assurance efforts. With a data revolution happening all around us, where does your business fit in? Are you struggling with valuable but unmanageable datasets? Or are you using data to propel your business into the future?
Unstructured Data Requires More Than Copy-PasteThere is no denying that the value of accurate, up-to-date, and consistent data for modern businesses is as vital as cloud computing and digital applications. Despite this reality, poor data quality still costs businesses an average of $13 million per year.
EventMetaBeat 2022
MetaBeat will bring together thought leaders to advise on how metaverse technology will transform the way all industries communicate and do business on October 4 in San Francisco, CA.
register hereTo manage data issues, you can apply statistical methods to measure data shapes, allowing your data teams to track variability, eliminate outliers, and reduce data drift. Statistics-based checks remain valuable for judging data quality and determining how and when you should turn to datasets before making critical decisions. Although effective, this statistical approach is generally reserved for structured data sets, which lend themselves to objective quantitative measurements.
But what about data that doesn't fit perfectly into Microsoft Excel or Google Sheets, including:
Internet of Things (IoT): Sensor Data, Ticker Data, and Log Data Multimedia: Photos, audio and videos Rich media: geospatial data, satellite imagery, weather data and monitoring data Documents: word processing documents, spreadsheets, presentations, emails and communication dataWhen these types of unstructured data are in play, it's easy for incomplete or inaccurate information to creep into the models. When errors go unnoticed, data problems accumulate and wreak havoc on everything from quarterly reports to forecast projections. A simple copy-and-paste approach from structured data to unstructured data is not enough and can actually make things worse for your business.
The common adage, "garbage in, garbage out", applies perfectly to unstructured datasets. It may be time to trash your current approach to data.
The Do's and Don'ts of Applying ML to Data Quality AssuranceWhen considering solutions for unstructured data, ML should be at the top of your list. That's because ML can analyze large data sets and quickly find patterns among the clutter — and with the right training, ML models can learn to interpret, organize, and...

Couldn't attend Transform 2022? Check out all the summit sessions in our on-demand library now! Look here.
We are in the midst of a data revolution. The volume of digital data created over the next five years will total double the amount produced so far – and unstructured data will define this new era of digital experiences.
Unstructured data (information that does not follow conventional patterns or fit into structured database formats) accounts for more than 80% of all new enterprise data. To prepare for this change, companies are finding innovative ways to manage, analyze and maximize the use of data in everything from business analytics to artificial intelligence (AI). But decision makers also face an age-old problem: how to maintain and improve the quality of large, unwieldy datasets?
With machine learning (ML), that's how it is. Advances in ML technology now allow organizations to efficiently process unstructured data and improve quality assurance efforts. With a data revolution happening all around us, where does your business fit in? Are you struggling with valuable but unmanageable datasets? Or are you using data to propel your business into the future?
Unstructured Data Requires More Than Copy-PasteThere is no denying that the value of accurate, up-to-date, and consistent data for modern businesses is as vital as cloud computing and digital applications. Despite this reality, poor data quality still costs businesses an average of $13 million per year.
EventMetaBeat 2022
MetaBeat will bring together thought leaders to advise on how metaverse technology will transform the way all industries communicate and do business on October 4 in San Francisco, CA.
register hereTo manage data issues, you can apply statistical methods to measure data shapes, allowing your data teams to track variability, eliminate outliers, and reduce data drift. Statistics-based checks remain valuable for judging data quality and determining how and when you should turn to datasets before making critical decisions. Although effective, this statistical approach is generally reserved for structured data sets, which lend themselves to objective quantitative measurements.
But what about data that doesn't fit perfectly into Microsoft Excel or Google Sheets, including:
Internet of Things (IoT): Sensor Data, Ticker Data, and Log Data Multimedia: Photos, audio and videos Rich media: geospatial data, satellite imagery, weather data and monitoring data Documents: word processing documents, spreadsheets, presentations, emails and communication dataWhen these types of unstructured data are in play, it's easy for incomplete or inaccurate information to creep into the models. When errors go unnoticed, data problems accumulate and wreak havoc on everything from quarterly reports to forecast projections. A simple copy-and-paste approach from structured data to unstructured data is not enough and can actually make things worse for your business.
The common adage, "garbage in, garbage out", applies perfectly to unstructured datasets. It may be time to trash your current approach to data.
The Do's and Don'ts of Applying ML to Data Quality AssuranceWhen considering solutions for unstructured data, ML should be at the top of your list. That's because ML can analyze large data sets and quickly find patterns among the clutter — and with the right training, ML models can learn to interpret, organize, and...
What's Your Reaction?
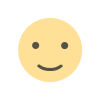
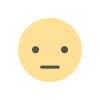
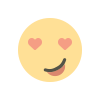
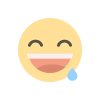
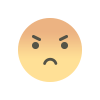
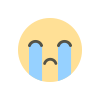
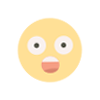