Two years after the debut of DALL-E, its inventor is "surprised" by the impact
Check out all the Smart Security Summit on-demand sessions here.
Before DALL-E 2, Stable Diffusion and Midjourney, there was only one research paper called "Zero-Shot Text-to-Image Generation".
With this article and a controlled website demo, on January 5, 2021 (two years ago today) OpenAI introduced DALL-E, a neural network that "creates images from text captions for a wide range of concepts expressible in natural language.”
The 12 billion parameter version of the Transformer GPT-3 language model was trained to generate images from text descriptions, using a dataset of text-image pairs. VentureBeat reporter Khari Johnson described the name as "intended to evoke artist Salvador Dali and the robot WALL-E" and included a DALL-E-generated illustration of a "baby daikon radish in a tutu walking around a dog".
Since then, things have moved rapidly, according to Aditya Ramesh, OpenAI researcher, inventor of DALL-E and co-inventor of DALL-E 2. That's more than an understatement, given the dizzying pace of development in the generative AI space over the past year. Then there was the meteoric rise of Diffusion models, which was a game-changer for DALL-E 2, released last April, and its open source counterparts, Stable Diffusion and Midjourney.
EventOn-Demand Smart Security Summit
Learn about the essential role of AI and ML in cybersecurity and industry-specific case studies. Watch the on-demand sessions today.
look here“Not so long ago, we felt like we were trying this direction of research to see what could be done,” Ramesh told VentureBeat. "I knew the technology was going to get to a point where it would have an impact on consumers and be useful for many different applications, but I was still surprised by the speed."
Now, generative modeling is approaching the point where "there will be a kind of iPhone-like moment for image generation and other modalities," he said. "I'm excited to be able to create something that will be used for all of these applications that will emerge."
DALL-E 1 research was developed and announced in conjunction with CLIP (Contrastive Language-Image Pre-training), a distinct model based on zero-shot learning that was essentially DALL-E's secret sauce. Trained on 400 million image pairs with text captions pulled from the internet, CLIP wa...

Check out all the Smart Security Summit on-demand sessions here.
Before DALL-E 2, Stable Diffusion and Midjourney, there was only one research paper called "Zero-Shot Text-to-Image Generation".
With this article and a controlled website demo, on January 5, 2021 (two years ago today) OpenAI introduced DALL-E, a neural network that "creates images from text captions for a wide range of concepts expressible in natural language.”
The 12 billion parameter version of the Transformer GPT-3 language model was trained to generate images from text descriptions, using a dataset of text-image pairs. VentureBeat reporter Khari Johnson described the name as "intended to evoke artist Salvador Dali and the robot WALL-E" and included a DALL-E-generated illustration of a "baby daikon radish in a tutu walking around a dog".
Since then, things have moved rapidly, according to Aditya Ramesh, OpenAI researcher, inventor of DALL-E and co-inventor of DALL-E 2. That's more than an understatement, given the dizzying pace of development in the generative AI space over the past year. Then there was the meteoric rise of Diffusion models, which was a game-changer for DALL-E 2, released last April, and its open source counterparts, Stable Diffusion and Midjourney.
EventOn-Demand Smart Security Summit
Learn about the essential role of AI and ML in cybersecurity and industry-specific case studies. Watch the on-demand sessions today.
look here“Not so long ago, we felt like we were trying this direction of research to see what could be done,” Ramesh told VentureBeat. "I knew the technology was going to get to a point where it would have an impact on consumers and be useful for many different applications, but I was still surprised by the speed."
Now, generative modeling is approaching the point where "there will be a kind of iPhone-like moment for image generation and other modalities," he said. "I'm excited to be able to create something that will be used for all of these applications that will emerge."
DALL-E 1 research was developed and announced in conjunction with CLIP (Contrastive Language-Image Pre-training), a distinct model based on zero-shot learning that was essentially DALL-E's secret sauce. Trained on 400 million image pairs with text captions pulled from the internet, CLIP wa...
What's Your Reaction?
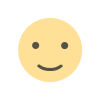
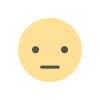
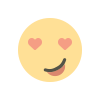
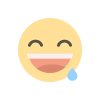
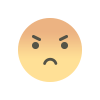
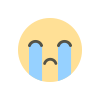
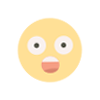