Waymo Collision Avoidance Test

Back to all posts
December 14, 2022
, TechnologyWe assess how well the Waymo Driver prevents crashes and mitigates the risk of serious injury in emergency situations by comparing its behavior to the behavior of a reference model of a non-disabled human driver, with eyes always on conflict (NIEON) - essentially, an attentive driver who doesn't get distracted or tired* - that we featured earlier this year. All human drivers occasionally take their eyes or attention away from the road. Thus, the NIEON model represents a level of performance that does not exist in the human population and provides a high benchmark against which to compare the Waymo driver.
To identify relevant test scenarios, we use existing driving data from Waymo's many years of experience, human accident data such as police accident databases and crashes recorded by cameras dashboard, and expert knowledge of our operational design domain, which includes geographies, driving conditions, and road types where our driver will be operating. Over time, we continue to add new and representative scenarios that we encounter on public roads and in simulations, or as we expand into new territories.Developed since 2016 and informed by our millions of miles traveled on public roads as well as thousands of real human crashes, our scenario database provides comprehensive coverage of dangerous situations. Because the most common crash types are similar no matter where you drive, our database can be used as a benchmark for any city, allowing for faster scalability. It contains a wide range of common situations that can occur almost anywhere, such as a car pulling out of a driveway or a pedestrian crossing against the signal.
The continuous search for scenarios is facilitated by the collection of data in new territories where the Waymo driver operates. For example, our driving in San Francisco...
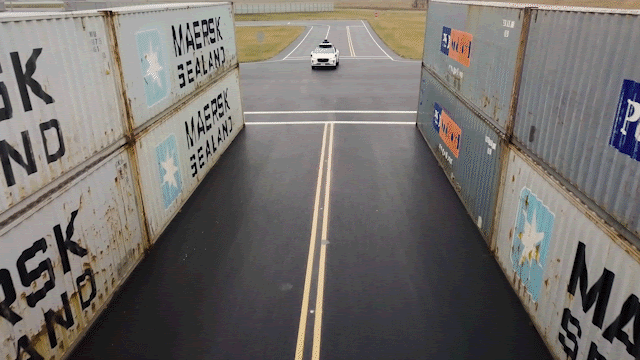
December 14, 2022
, TechnologyWe assess how well the Waymo Driver prevents crashes and mitigates the risk of serious injury in emergency situations by comparing its behavior to the behavior of a reference model of a non-disabled human driver, with eyes always on conflict (NIEON) - essentially, an attentive driver who doesn't get distracted or tired* - that we featured earlier this year. All human drivers occasionally take their eyes or attention away from the road. Thus, the NIEON model represents a level of performance that does not exist in the human population and provides a high benchmark against which to compare the Waymo driver.
To identify relevant test scenarios, we use existing driving data from Waymo's many years of experience, human accident data such as police accident databases and crashes recorded by cameras dashboard, and expert knowledge of our operational design domain, which includes geographies, driving conditions, and road types where our driver will be operating. Over time, we continue to add new and representative scenarios that we encounter on public roads and in simulations, or as we expand into new territories.Developed since 2016 and informed by our millions of miles traveled on public roads as well as thousands of real human crashes, our scenario database provides comprehensive coverage of dangerous situations. Because the most common crash types are similar no matter where you drive, our database can be used as a benchmark for any city, allowing for faster scalability. It contains a wide range of common situations that can occur almost anywhere, such as a car pulling out of a driveway or a pedestrian crossing against the signal.
The continuous search for scenarios is facilitated by the collection of data in new territories where the Waymo driver operates. For example, our driving in San Francisco...
What's Your Reaction?
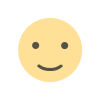
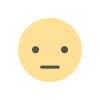
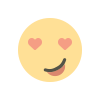
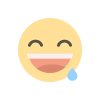
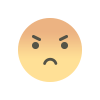
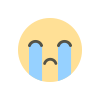
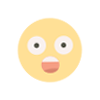