3 essential capabilities missing from the AI
Couldn't attend Transform 2022? Check out all the summit sessions in our on-demand library now! Look here.
Over the past decade, deep learning has come a long way, growing from a promising area of artificial intelligence (AI) research to a mainstay of many applications. However, despite advances in deep learning, some of its problems have not gone away. Among them are three essential abilities: understanding concepts, forming abstractions and making analogies, according to Melanie Mitchell, professor at the Santa Fe Institute and author of "Artificial Intelligence: A Guide for Thinking Humans".
In a recent seminar at the Institute of Advanced Research in Artificial Intelligence, Mitchell explained why abstraction and analogy are keys to creating robust AI systems. While the notion of abstraction has been around since the term "artificial intelligence" was coined in 1955, this field has remained largely understudied, says Mitchell.
As the AI community increasingly places importance and resources on data-driven approaches and deep learning, Mitchell warns that what appears to be human-like performance by networks of neurons is, in fact, a superficial imitation that misses the key components of intelligence.
From concepts to analogies"There are many different definitions of 'concept' in the cognitive science literature, but I particularly like Lawrence Barsalou's: a concept is 'a skill or disposition to generate infinite conceptualizations of a category '" Mitchell told VentureBeat. .
EventMetaBeat 2022
MetaBeat will bring together thought leaders to advise on how metaverse technology will transform the way all industries communicate and do business on October 4 in San Francisco, CA.
register hereFor example, when we think of a category like "trees", we can think of all kinds of different trees, both real and imaginary, realistic or cartoonish, concrete or metaphorical. We can think of natural trees, genealogical trees or organizational trees.
"There is some essential similarity - call it 'tree' - between all of these," Mitchell said. "Essentially, a concept is a generative mental model that is part of a large web of other concepts."
While scientists and AI researchers often refer to neural networks as learning concepts, the main difference Mitchell points out is what these computer architectures learn. While humans create "generative" models that can form abstractions and use them in novel ways, deep learning systems are "discriminative" models that can only learn superficial differences between different categories.
For example, a deep learning model trained on many labeled images of bridges will be able to detect new bridges, but it will not be able to examine other elements based on the same concept, such as a log connecting two river banks or ants forming a bridge to bridge a void, or abstract notions of "bridge", such as bridging a social gap.
Discriminator models have predefined categories that the system can choose from: for example, does the photo represent a dog, a cat or a coyote? Rather, to flexibly apply his knowledge to a new situation, Mitchell explained.
"You have to generate an analogy - for example, if I know something about trees, and I see a picture of a human lung, with all its branching structure, I don't classify it as a tree, but I recognize similarities on an abstract level - I take what I know and map it to a new situation,” she said.
Why is this important? The real world is full of never-before-seen situations. It is important to learn from as...

Couldn't attend Transform 2022? Check out all the summit sessions in our on-demand library now! Look here.
Over the past decade, deep learning has come a long way, growing from a promising area of artificial intelligence (AI) research to a mainstay of many applications. However, despite advances in deep learning, some of its problems have not gone away. Among them are three essential abilities: understanding concepts, forming abstractions and making analogies, according to Melanie Mitchell, professor at the Santa Fe Institute and author of "Artificial Intelligence: A Guide for Thinking Humans".
In a recent seminar at the Institute of Advanced Research in Artificial Intelligence, Mitchell explained why abstraction and analogy are keys to creating robust AI systems. While the notion of abstraction has been around since the term "artificial intelligence" was coined in 1955, this field has remained largely understudied, says Mitchell.
As the AI community increasingly places importance and resources on data-driven approaches and deep learning, Mitchell warns that what appears to be human-like performance by networks of neurons is, in fact, a superficial imitation that misses the key components of intelligence.
From concepts to analogies"There are many different definitions of 'concept' in the cognitive science literature, but I particularly like Lawrence Barsalou's: a concept is 'a skill or disposition to generate infinite conceptualizations of a category '" Mitchell told VentureBeat. .
EventMetaBeat 2022
MetaBeat will bring together thought leaders to advise on how metaverse technology will transform the way all industries communicate and do business on October 4 in San Francisco, CA.
register hereFor example, when we think of a category like "trees", we can think of all kinds of different trees, both real and imaginary, realistic or cartoonish, concrete or metaphorical. We can think of natural trees, genealogical trees or organizational trees.
"There is some essential similarity - call it 'tree' - between all of these," Mitchell said. "Essentially, a concept is a generative mental model that is part of a large web of other concepts."
While scientists and AI researchers often refer to neural networks as learning concepts, the main difference Mitchell points out is what these computer architectures learn. While humans create "generative" models that can form abstractions and use them in novel ways, deep learning systems are "discriminative" models that can only learn superficial differences between different categories.
For example, a deep learning model trained on many labeled images of bridges will be able to detect new bridges, but it will not be able to examine other elements based on the same concept, such as a log connecting two river banks or ants forming a bridge to bridge a void, or abstract notions of "bridge", such as bridging a social gap.
Discriminator models have predefined categories that the system can choose from: for example, does the photo represent a dog, a cat or a coyote? Rather, to flexibly apply his knowledge to a new situation, Mitchell explained.
"You have to generate an analogy - for example, if I know something about trees, and I see a picture of a human lung, with all its branching structure, I don't classify it as a tree, but I recognize similarities on an abstract level - I take what I know and map it to a new situation,” she said.
Why is this important? The real world is full of never-before-seen situations. It is important to learn from as...
What's Your Reaction?
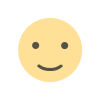
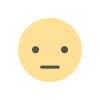
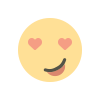
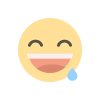
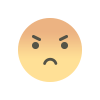
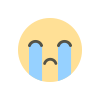
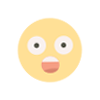