Create Responsible AI Products Using Human Supervision
We're excited to bring Transform 2022 back in person on July 19 and virtually from July 20-28. Join leaders in AI and data for in-depth discussions and exciting networking opportunities. Sign up today!
As a business, you no longer need to develop everything from scratch or train your own ML models. With machine learning as a service (MLaaS) becoming more ubiquitous, the market is flooded with many turnkey solutions and ML platforms. According to Mordor Intelligence, the market is expected to reach $17 billion by 2027.
The marketTotal funding for AI startups worldwide was nearly $40 billion last year, up from less than $1 billion a decade ago. Many large and small cloud companies that have entered the MLOps space are now beginning to realize the need for human involvement when operating their models.
The main goal of many AI platforms is to become attractive to the general user by making ML widely automated and available in low-code environments. But whether companies build ML solutions exclusively for their own use or for the benefit of their customers, there is a common problem: many of them train and monitor their models on poor quality data. Models trained on these types of data can produce predictions and therefore products that are inherently biased, misleading, and ultimately of inferior quality.
Models and human involvementMany of them are encoder-decoder models that use recurrent neural networks for sequence-to-sequence prediction. They work by taking an input, converting it into a vector, and then decoding it into a sentence; a similar approach works if the initial input is, say, an image. These have a wide range of applications - from virtual assistants to content moderation.
EventTransform 2022
Join us at the leading Applied AI event for enterprise business and technology decision makers on July 19 and virtually July 20-28.
register hereThe problem is that human-managed data is often used haphazardly and without proper oversight to support these models, which can lead to multiple problems down the road. However, these patterns are part of the larger human-in-the-loop framework, i.e., they involve human interaction by design. With this in mind, they must be under constant oversight at every stage of production to enable responsible AI products. But what exactly does it mean for an AI product to be "responsible"?
What is Responsible AI?According to most AI researchers, the notion of responsible AI is about improving the lives of people around the world by "always considering the ethical and societal implications". ...

We're excited to bring Transform 2022 back in person on July 19 and virtually from July 20-28. Join leaders in AI and data for in-depth discussions and exciting networking opportunities. Sign up today!
As a business, you no longer need to develop everything from scratch or train your own ML models. With machine learning as a service (MLaaS) becoming more ubiquitous, the market is flooded with many turnkey solutions and ML platforms. According to Mordor Intelligence, the market is expected to reach $17 billion by 2027.
The marketTotal funding for AI startups worldwide was nearly $40 billion last year, up from less than $1 billion a decade ago. Many large and small cloud companies that have entered the MLOps space are now beginning to realize the need for human involvement when operating their models.
The main goal of many AI platforms is to become attractive to the general user by making ML widely automated and available in low-code environments. But whether companies build ML solutions exclusively for their own use or for the benefit of their customers, there is a common problem: many of them train and monitor their models on poor quality data. Models trained on these types of data can produce predictions and therefore products that are inherently biased, misleading, and ultimately of inferior quality.
Models and human involvementMany of them are encoder-decoder models that use recurrent neural networks for sequence-to-sequence prediction. They work by taking an input, converting it into a vector, and then decoding it into a sentence; a similar approach works if the initial input is, say, an image. These have a wide range of applications - from virtual assistants to content moderation.
EventTransform 2022
Join us at the leading Applied AI event for enterprise business and technology decision makers on July 19 and virtually July 20-28.
register hereThe problem is that human-managed data is often used haphazardly and without proper oversight to support these models, which can lead to multiple problems down the road. However, these patterns are part of the larger human-in-the-loop framework, i.e., they involve human interaction by design. With this in mind, they must be under constant oversight at every stage of production to enable responsible AI products. But what exactly does it mean for an AI product to be "responsible"?
What is Responsible AI?According to most AI researchers, the notion of responsible AI is about improving the lives of people around the world by "always considering the ethical and societal implications". ...
What's Your Reaction?
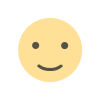
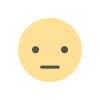
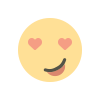
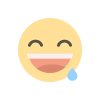
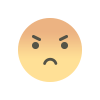
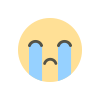
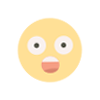