Detect harmful gases with a single sensor and tinyML

A chemical and/or gas leak can be potentially fatal to people and the environment, which is why it is vital to detect them as soon as possible. But instead of relying on simple thresholds, Roni Bandini was able to develop a system that could detect custom leaks by recognizing subtle changes in gas level values using machine learning.
To do this, Bandini took a single MiCS-4514 and connected it to an Arduino Nano 33 BLE Sense, complete with an OLED display, a fan, and a buzzer to send alerts. The MiCS-4514 is a multi-gas sensor capable of detecting methane, ethanol, hydrogen, ammonia, carbon monoxide and nitrogen dioxide. This capability means that explosive and/or toxic gases can be identified long before they build up to a critical level inside.

Once multiple samples were collected, ranging from typical to dangerous levels, Bandini fed the dataset into Edge Impulse Studio to train a neural network classifier on the time series samples. Each time the device starts, the sensor is calibrated for a preset time and can be used to distinguish harmful air quality within 1.5 seconds. The screen displays any high sensor readings and if a leak has been detected.
To learn more about this project, you can read Bandini's tutorial or watch this demo video below.


A chemical and/or gas leak can be potentially fatal to people and the environment, which is why it is vital to detect them as soon as possible. But instead of relying on simple thresholds, Roni Bandini was able to develop a system that could detect custom leaks by recognizing subtle changes in gas level values using machine learning.
To do this, Bandini took a single MiCS-4514 and connected it to an Arduino Nano 33 BLE Sense, complete with an OLED display, a fan, and a buzzer to send alerts. The MiCS-4514 is a multi-gas sensor capable of detecting methane, ethanol, hydrogen, ammonia, carbon monoxide and nitrogen dioxide. This capability means that explosive and/or toxic gases can be identified long before they build up to a critical level inside.

Once multiple samples were collected, ranging from typical to dangerous levels, Bandini fed the dataset into Edge Impulse Studio to train a neural network classifier on the time series samples. Each time the device starts, the sensor is calibrated for a preset time and can be used to distinguish harmful air quality within 1.5 seconds. The screen displays any high sensor readings and if a leak has been detected.
To learn more about this project, you can read Bandini's tutorial or watch this demo video below.
What's Your Reaction?
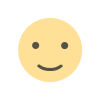
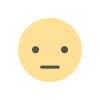
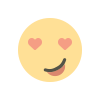
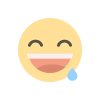
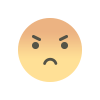
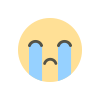
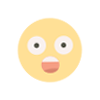