Transform 2022: How enterprises explore, walk, then collide with their AI/ML deployments
We're excited to bring Transform 2022 back in person on July 19 and virtually from July 20-28. Join leaders in AI and data for in-depth discussions and exciting networking opportunities. Sign up today!
SAN FRANCISCO – Businesses don't resist AI/ML deployments overnight, and when the decision is made to do so, it involves most of the company's executives and many analyst hires qualified and scientific data. It also involves an evolution that can be compared to a person learning to crawl, walk and then run.
None of this is easy or simple, but it becomes necessary in the third decade of the 21st century. Businesses are learning to crawl, walk and run when it comes to using their data to give them deeper insight into their protected business data, all the superfluous data that sits in storage vaults but that are not accounted for, and all their historical data. Do not forget all the social networks and external data (customer reviews, product reviews, etc.) that float in the gigantic universe that is the Internet and affect a company from near or far.
At VentureBeat's Transform 2022 conference here at the Palace Hotel, a panel consisting of Fiona Tan, CTO of Wayfair; Rajat Shroff, VP of Product, DoorDash; Kevin Zielnicki, Senior Data Scientist, Stitch Fix; and moderator Sharon Goldman, Editor and Writer, VentureBeat, discussed how their automated AI/ML processes help scale and accelerate to market. Their journeys have taken them all from proof of concept to production in a sustainable way.
DoorDash's approach“At DoorDash, one of our values is that we dream big but start small,” Shroff said. “We also apply this to our AI efforts. We will start by using manual means to do non-scalable things to learn and understand how to find a suitable product for the market. Once we see the signal, that's when we start inventing algorithms and scaling them.
EventTransform 2022
Join us at the leading Applied AI event for enterprise business and technology decision makers on July 19 and virtually July 20-28.
register here“For example, when we did our analytics, we found that only about 8% of our business delivered pizza. Some of us thought that was maybe half of our business. realized that we needed to be much more accurate in our assessments, so we got the team together and said, "We need to get to 99% accuracy." After a few months of manually annotating the data collection, the team found a small sample (identifying a market, a category). Once they got a signal, they expanded the whole project. Once they got to a level of precision they liked , that's when they passed it to the ML team. And they started building (AI models)."
After a few months of team building and deployment, DoorDash went from 60% accuracy in analyzing its business to its goal of 99%, Shroff said.
How Wayfair uses AI/ML“We started our (IA) project by looking at the accessibility and quality of data available for the problems we were trying to solve,” Tan said, “so we wanted to make sure we had the ingredients to apply to our AI/ML project. The second consideration we wanted to know was "How much tolerance do we have for wrong predictions?" So the first place we decided to go with our project was in areas within

We're excited to bring Transform 2022 back in person on July 19 and virtually from July 20-28. Join leaders in AI and data for in-depth discussions and exciting networking opportunities. Sign up today!
SAN FRANCISCO – Businesses don't resist AI/ML deployments overnight, and when the decision is made to do so, it involves most of the company's executives and many analyst hires qualified and scientific data. It also involves an evolution that can be compared to a person learning to crawl, walk and then run.
None of this is easy or simple, but it becomes necessary in the third decade of the 21st century. Businesses are learning to crawl, walk and run when it comes to using their data to give them deeper insight into their protected business data, all the superfluous data that sits in storage vaults but that are not accounted for, and all their historical data. Do not forget all the social networks and external data (customer reviews, product reviews, etc.) that float in the gigantic universe that is the Internet and affect a company from near or far.
At VentureBeat's Transform 2022 conference here at the Palace Hotel, a panel consisting of Fiona Tan, CTO of Wayfair; Rajat Shroff, VP of Product, DoorDash; Kevin Zielnicki, Senior Data Scientist, Stitch Fix; and moderator Sharon Goldman, Editor and Writer, VentureBeat, discussed how their automated AI/ML processes help scale and accelerate to market. Their journeys have taken them all from proof of concept to production in a sustainable way.
DoorDash's approach“At DoorDash, one of our values is that we dream big but start small,” Shroff said. “We also apply this to our AI efforts. We will start by using manual means to do non-scalable things to learn and understand how to find a suitable product for the market. Once we see the signal, that's when we start inventing algorithms and scaling them.
EventTransform 2022
Join us at the leading Applied AI event for enterprise business and technology decision makers on July 19 and virtually July 20-28.
register here“For example, when we did our analytics, we found that only about 8% of our business delivered pizza. Some of us thought that was maybe half of our business. realized that we needed to be much more accurate in our assessments, so we got the team together and said, "We need to get to 99% accuracy." After a few months of manually annotating the data collection, the team found a small sample (identifying a market, a category). Once they got a signal, they expanded the whole project. Once they got to a level of precision they liked , that's when they passed it to the ML team. And they started building (AI models)."
After a few months of team building and deployment, DoorDash went from 60% accuracy in analyzing its business to its goal of 99%, Shroff said.
How Wayfair uses AI/ML“We started our (IA) project by looking at the accessibility and quality of data available for the problems we were trying to solve,” Tan said, “so we wanted to make sure we had the ingredients to apply to our AI/ML project. The second consideration we wanted to know was "How much tolerance do we have for wrong predictions?" So the first place we decided to go with our project was in areas within
What's Your Reaction?
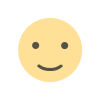
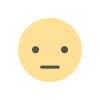
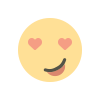
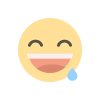
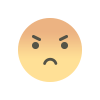
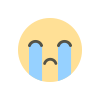
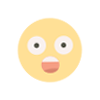