Prevent your public cloud AI projects from drying up
Check out the on-demand sessions from the Low-Code/No-Code Summit to learn how to successfully innovate and gain efficiencies by improving and scaling citizen developers. Watch now.
Last year, Andreessen Horowitz published a provocative blog post titled “The Cost of the Cloud, A Trillion-Dollar Paradox.” In it, the venture capital firm argued that runaway cloud spending is resulting in public companies leaving billions of dollars in potential market capitalization on the table. An alternative, the company suggests, is to recalibrate cloud resources into a hybrid model. Such a model can boost a company's bottom line and free up capital to focus on new products and growth.
It remains to be seen whether companies will follow this advice, but one thing is certain: CIOs demand more agility and performance from their support infrastructure. This is especially true when looking to use sophisticated and computationally intensive artificial intelligence/machine learning (AI/ML) applications to improve their ability to make real-time, data-driven decisions.
To this end, the public cloud has played a fundamental role in bringing AI into the mainstream. But the factors that have made the public cloud an ideal proving ground for AI (i.e. elastic pricing, ease of flexing up or down, among other factors) actually prevent AI to realize its full potential.
Here are some considerations for organizations looking to maximize the benefits of AI in their environments.
EventSmart Security Summit
Learn about the essential role of AI and ML in cybersecurity and industry-specific case studies on December 8. Sign up for your free pass today.
Register now For AI, the cloud is not uniqueData is the lifeblood of modern business, the fuel that drives AI insights. And since many AI workloads must constantly ingest large and growing volumes of data, it is imperative that the infrastructure can meet these demands in a cost-effective and efficient manner.
When deciding how best to approach AI at scale, IT leaders need to consider a variety of factors. The first is whether colocation, public cloud, or a hybrid mix is best suited to meet the unique needs of modern AI applications.
While the public cloud has played an invaluable role in bringing AI to market, it comes with its own set of challenges. These include:
Vendor lock-in: Most cloud-based services have some risk of lock-in. However, some cloud-based AI services available today are very platform-specific, each sporting their own particular nuances and distinct partner-related integrations. Consequently, many organizations tend to consolidate their AI workloads with a single vendor. This makes it difficult for them to switch suppliers in the future without incurring significant costs. Elastic Pricing: The ability to pay only for what you use is what makes public cloud such an attractive option for enterprises, especially those hoping to reduce their CapEx spend. And consuming a public cloud service bit by bit often makes economic sense in the short term. But organizations with limited visibility into their cloud usage too often find they're consuming it by the bucketful. At this point, it becomes a tax that stifles innovation. Egress charges: With cloud data transfers, a customer does not need to pay for the data they send to the cloud. But to pull that data from the cloud, they have to pay egress fees, which can quickly add up. For example, disaster recovery systems will often be spread across multiple geographic regions to ensure resilience...
Check out the on-demand sessions from the Low-Code/No-Code Summit to learn how to successfully innovate and gain efficiencies by improving and scaling citizen developers. Watch now.
Last year, Andreessen Horowitz published a provocative blog post titled “The Cost of the Cloud, A Trillion-Dollar Paradox.” In it, the venture capital firm argued that runaway cloud spending is resulting in public companies leaving billions of dollars in potential market capitalization on the table. An alternative, the company suggests, is to recalibrate cloud resources into a hybrid model. Such a model can boost a company's bottom line and free up capital to focus on new products and growth.
It remains to be seen whether companies will follow this advice, but one thing is certain: CIOs demand more agility and performance from their support infrastructure. This is especially true when looking to use sophisticated and computationally intensive artificial intelligence/machine learning (AI/ML) applications to improve their ability to make real-time, data-driven decisions.
To this end, the public cloud has played a fundamental role in bringing AI into the mainstream. But the factors that have made the public cloud an ideal proving ground for AI (i.e. elastic pricing, ease of flexing up or down, among other factors) actually prevent AI to realize its full potential.
Here are some considerations for organizations looking to maximize the benefits of AI in their environments.
EventSmart Security Summit
Learn about the essential role of AI and ML in cybersecurity and industry-specific case studies on December 8. Sign up for your free pass today.
Register now For AI, the cloud is not uniqueData is the lifeblood of modern business, the fuel that drives AI insights. And since many AI workloads must constantly ingest large and growing volumes of data, it is imperative that the infrastructure can meet these demands in a cost-effective and efficient manner.
When deciding how best to approach AI at scale, IT leaders need to consider a variety of factors. The first is whether colocation, public cloud, or a hybrid mix is best suited to meet the unique needs of modern AI applications.
While the public cloud has played an invaluable role in bringing AI to market, it comes with its own set of challenges. These include:
Vendor lock-in: Most cloud-based services have some risk of lock-in. However, some cloud-based AI services available today are very platform-specific, each sporting their own particular nuances and distinct partner-related integrations. Consequently, many organizations tend to consolidate their AI workloads with a single vendor. This makes it difficult for them to switch suppliers in the future without incurring significant costs. Elastic Pricing: The ability to pay only for what you use is what makes public cloud such an attractive option for enterprises, especially those hoping to reduce their CapEx spend. And consuming a public cloud service bit by bit often makes economic sense in the short term. But organizations with limited visibility into their cloud usage too often find they're consuming it by the bucketful. At this point, it becomes a tax that stifles innovation. Egress charges: With cloud data transfers, a customer does not need to pay for the data they send to the cloud. But to pull that data from the cloud, they have to pay egress fees, which can quickly add up. For example, disaster recovery systems will often be spread across multiple geographic regions to ensure resilience...What's Your Reaction?
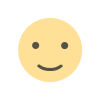
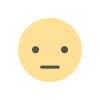
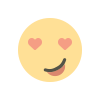
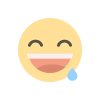
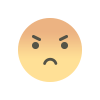
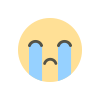
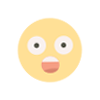