Why composability is key to scaling digital twins
Couldn't attend Transform 2022? Check out all the summit sessions in our on-demand library now! Look here.
Digital twins allow companies to model and simulate buildings, products, manufacturing lines, facilities and processes. This can improve performance, flag quality errors quickly, and support better decision making. Today, most digital twin projects are one-time efforts. A team can create a digital twin for a new gearbox and start all over again when modeling a wind turbine that includes that part or the business process that repairs that part.
Ideally, engineers would like to quickly assemble more complex digital twins to represent turbines, wind farms, power grids, and energy companies. This is complicated by the various components that go into digital twins beyond the physical models, such as data management, semantic labels, security, and user interface (UI). New approaches to composing digital elements into larger assemblies and models could help simplify this process.
Gartner has predicted that the digital twin market will cross the chasm in 2026 to reach $183 billion by 2031, with composite digital twins presenting the greatest opportunity. He recommends product managers create ecosystems and libraries of prebuilt functions and vertical market templates to drive competitiveness in the digital twin market. The industry is starting to take notice.
The Digital Twin Consortium recently released the Capability Periodic Table (CPT) framework to help organizations develop composable digital twins. It organizes the supporting technology landscape to help teams create the foundation for integrating individual digital twins.
EventMetaBeat 2022
MetaBeat will bring together thought leaders to advise on how metaverse technology will transform the way all industries communicate and do business on October 4 in San Francisco, CA.
register here A new type of modelImportant similarities and differences exist in the modeling used to create digital twins compared to other analytics and artificial intelligence (AI) models. All of these efforts begin with appropriate and timely historical data to inform model design and calibrate the current state with model results.
However, digital twin simulations are unique from traditional statistical learning approaches in that the structures of the model are not learned directly from the data, said Bret Greenstein, data partner, analytics and AI at PwC, at VentureBeat. Instead, a model structure is presented by modellers through interviews, research and design sessions with domain experts to align with strategic or operational questions that are defined in advance. /p>
Therefore, domain experts should be involved in informing and validating the structure of the model. This time investment can limit the scope of simulations to applications requiring continuous scenario analysis. Greenstein also finds that developing a digital twin model is an ongoing exercise. The granularity of the model and the boundaries of the systems must be carefully considered and defined to balance the investment of time and the suitability of the model for the questions they are intended to support.
"If organizations are unable to effectively attract...

Couldn't attend Transform 2022? Check out all the summit sessions in our on-demand library now! Look here.
Digital twins allow companies to model and simulate buildings, products, manufacturing lines, facilities and processes. This can improve performance, flag quality errors quickly, and support better decision making. Today, most digital twin projects are one-time efforts. A team can create a digital twin for a new gearbox and start all over again when modeling a wind turbine that includes that part or the business process that repairs that part.
Ideally, engineers would like to quickly assemble more complex digital twins to represent turbines, wind farms, power grids, and energy companies. This is complicated by the various components that go into digital twins beyond the physical models, such as data management, semantic labels, security, and user interface (UI). New approaches to composing digital elements into larger assemblies and models could help simplify this process.
Gartner has predicted that the digital twin market will cross the chasm in 2026 to reach $183 billion by 2031, with composite digital twins presenting the greatest opportunity. He recommends product managers create ecosystems and libraries of prebuilt functions and vertical market templates to drive competitiveness in the digital twin market. The industry is starting to take notice.
The Digital Twin Consortium recently released the Capability Periodic Table (CPT) framework to help organizations develop composable digital twins. It organizes the supporting technology landscape to help teams create the foundation for integrating individual digital twins.
EventMetaBeat 2022
MetaBeat will bring together thought leaders to advise on how metaverse technology will transform the way all industries communicate and do business on October 4 in San Francisco, CA.
register here A new type of modelImportant similarities and differences exist in the modeling used to create digital twins compared to other analytics and artificial intelligence (AI) models. All of these efforts begin with appropriate and timely historical data to inform model design and calibrate the current state with model results.
However, digital twin simulations are unique from traditional statistical learning approaches in that the structures of the model are not learned directly from the data, said Bret Greenstein, data partner, analytics and AI at PwC, at VentureBeat. Instead, a model structure is presented by modellers through interviews, research and design sessions with domain experts to align with strategic or operational questions that are defined in advance. /p>
Therefore, domain experts should be involved in informing and validating the structure of the model. This time investment can limit the scope of simulations to applications requiring continuous scenario analysis. Greenstein also finds that developing a digital twin model is an ongoing exercise. The granularity of the model and the boundaries of the systems must be carefully considered and defined to balance the investment of time and the suitability of the model for the questions they are intended to support.
"If organizations are unable to effectively attract...
What's Your Reaction?
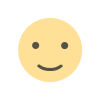
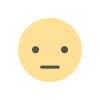
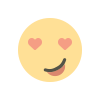
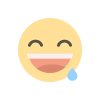
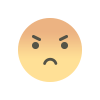
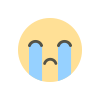
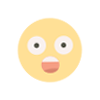