Why You Should Care About Computer Privacy Technologies
We're excited to bring Transform 2022 back in person on July 19 and virtually from July 20-28. Join leaders in AI and data for in-depth discussions and exciting networking opportunities. Sign up today!
In today's digital economy, data is the new oil. Businesses and organizations are hungry for access to new data, driving an ever-increasing demand for cross-organizational and cross-industry data collaborations. Unfortunately, data collaborations are being challenged by equally growing concerns about security, privacy and data privacy. This often prevents companies from extracting value from sensitive data and hinders the pace of innovation.
For example, the medical industry aspires to cross-industry data collaborations to advance medical research and drug discovery with the help of AI, but it also faces regulatory restrictions and legal issues related to patient privacy. Similarly, in banking, cross-agency data collaboration is essential to combat financial crimes, such as money laundering, but the cost of such collaboration is often prohibitive due to privacy regulations. and data privacy.
Wouldn't it be nice to have technology that could facilitate collaboration and data computation without ever revealing or compromising the underlying data? This is where privacy computing technologies (i.e. data itself) come in.
Gartner has ranked private computing as one of the top strategic technology trends in 2021. In this article, I will briefly discuss some of the approaches to private computing and share my perspective from the point of view of a high-tech VC.
EventTransform 2022
Join us at the leading Applied AI event for enterprise business and technology decision makers on July 19 and virtually July 20-28.
register here Multiparty calculationMPC is a software-based security protocol in which multiple data owners jointly compute a function on their individual inputs while keeping the input data private. Data security is achieved by mixing data from individual parties and distributing it to multiple parties for joint computations, all without the need to trust any of the parties (a.k.a. trustlessness).
Mathematically speaking, MPC is an elegant and secure approach, although there are some inherent problems in practical applications. For example, MPC calculations involve a lot of data exchange between parties and therefore can be vulnerable to network latency and are often limited by the slowest data link between parties. Many researchers are continuously improving MPC technology. Startups like Baffle and Inpher, to name a few, have managed to gain traction with practical use cases of MPC, especially in the finance and healthcare sectors.
Reliable execution environmentAnother important computing approach to privacy is TEE, sometimes referred to as a trusted enclave or confidential computing. TEE technology is a hardware solution that uses a secure area on a CPU t...

We're excited to bring Transform 2022 back in person on July 19 and virtually from July 20-28. Join leaders in AI and data for in-depth discussions and exciting networking opportunities. Sign up today!
In today's digital economy, data is the new oil. Businesses and organizations are hungry for access to new data, driving an ever-increasing demand for cross-organizational and cross-industry data collaborations. Unfortunately, data collaborations are being challenged by equally growing concerns about security, privacy and data privacy. This often prevents companies from extracting value from sensitive data and hinders the pace of innovation.
For example, the medical industry aspires to cross-industry data collaborations to advance medical research and drug discovery with the help of AI, but it also faces regulatory restrictions and legal issues related to patient privacy. Similarly, in banking, cross-agency data collaboration is essential to combat financial crimes, such as money laundering, but the cost of such collaboration is often prohibitive due to privacy regulations. and data privacy.
Wouldn't it be nice to have technology that could facilitate collaboration and data computation without ever revealing or compromising the underlying data? This is where privacy computing technologies (i.e. data itself) come in.
Gartner has ranked private computing as one of the top strategic technology trends in 2021. In this article, I will briefly discuss some of the approaches to private computing and share my perspective from the point of view of a high-tech VC.
EventTransform 2022
Join us at the leading Applied AI event for enterprise business and technology decision makers on July 19 and virtually July 20-28.
register here Multiparty calculationMPC is a software-based security protocol in which multiple data owners jointly compute a function on their individual inputs while keeping the input data private. Data security is achieved by mixing data from individual parties and distributing it to multiple parties for joint computations, all without the need to trust any of the parties (a.k.a. trustlessness).
Mathematically speaking, MPC is an elegant and secure approach, although there are some inherent problems in practical applications. For example, MPC calculations involve a lot of data exchange between parties and therefore can be vulnerable to network latency and are often limited by the slowest data link between parties. Many researchers are continuously improving MPC technology. Startups like Baffle and Inpher, to name a few, have managed to gain traction with practical use cases of MPC, especially in the finance and healthcare sectors.
Reliable execution environmentAnother important computing approach to privacy is TEE, sometimes referred to as a trusted enclave or confidential computing. TEE technology is a hardware solution that uses a secure area on a CPU t...
What's Your Reaction?
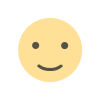
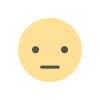
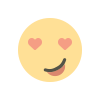
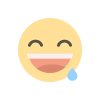
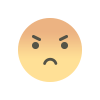
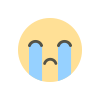
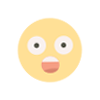