With “GitHub for data”, Gable.ai wants to connect software engineers and ML developers
Head to our on-demand library to view VB Transform 2023 sessions. Register here
AI applications are booming. But to prevent them from breaking, the data circulating in these applications must be of high quality, that is to say reliable, complete and precise.
That's the problem Gable.ai is poised to solve as the Seattle-based startup launches from stealth today with $7 million in seed funding. It calls its offering a premier data collaboration platform that enables software and data/ML developers to iteratively create and manage high-quality data assets, but investors have become accustomed to l call it “GitHub for data” – a solution that other data companies like Kaggle and Hex are investing in.
“GitHub really affects culture: it helps software engineers across the company communicate with each other much more effectively,” said Chad Sanderson, CEO and co-founder of Gable.ai. “But that doesn’t exist for data at all.”
Gable.ai's platform allows data producers and consumers to work together, he told VentureBeat. It helps software and data developers avoid abrupt changes to critical data workflows within their existing data infrastructure. The platform offers data asset recognition by connecting data sources; creating data contracts to establish ownership of data assets and define meaningful constraints; and enforcing data contracts through continuous integration/continuous deployment within GitHub.
EventVB Transform 2023 on demand
Did you miss a session of VB Transform 2023? Sign up to access the on-demand library for all of our featured sessions.
Register now The founders led the data department at ConvoyBefore founding Gable.ai, Sanderson and his co-founders, Adrian Kreuziger and Daniel Dicker, led the data department at Convoy, the $4 billion digital freight network that moves thousands of trucks every day across the country via an optimized and connected network. carrier network. Complex data was coming in fast and furious, regarding shipments, shippers, facilities, carriers, trucks, contracts and prices.
Although the company had a modern data stack, using the latest and greatest technologies, no one had confidence in the data: there were constant data quality issues, outages for valuable models and billions of rows of data could not be used. .
"When our data science team and analytics team were trying to understand even simple questions like 'How many shipments have we completed in the last 30 days?', all that complexity made it almost impossible to answer this question." » said Sanderson. “And it was the same problem with machine learning: the models were very, very sensitive and the data scientist had to figure out exactly what data from this very complex system needed to be fed into that model. When data quality was bad, when something suddenly changed, all these sensitive models started to break down and all the predictions they made turned out to be wrong. »
Ultimately, he explained, the problem was a lack of communication between software engineers and ML developers. “Once we helped close this gap, we saw an exponential improvement in data quality almost immediately,” he said.
In order to scale AI, it is essential to resolve communication issues related to data changes, Sanderson emphasized.
"If you don't have a change management system...

Head to our on-demand library to view VB Transform 2023 sessions. Register here
AI applications are booming. But to prevent them from breaking, the data circulating in these applications must be of high quality, that is to say reliable, complete and precise.
That's the problem Gable.ai is poised to solve as the Seattle-based startup launches from stealth today with $7 million in seed funding. It calls its offering a premier data collaboration platform that enables software and data/ML developers to iteratively create and manage high-quality data assets, but investors have become accustomed to l call it “GitHub for data” – a solution that other data companies like Kaggle and Hex are investing in.
“GitHub really affects culture: it helps software engineers across the company communicate with each other much more effectively,” said Chad Sanderson, CEO and co-founder of Gable.ai. “But that doesn’t exist for data at all.”
Gable.ai's platform allows data producers and consumers to work together, he told VentureBeat. It helps software and data developers avoid abrupt changes to critical data workflows within their existing data infrastructure. The platform offers data asset recognition by connecting data sources; creating data contracts to establish ownership of data assets and define meaningful constraints; and enforcing data contracts through continuous integration/continuous deployment within GitHub.
EventVB Transform 2023 on demand
Did you miss a session of VB Transform 2023? Sign up to access the on-demand library for all of our featured sessions.
Register now The founders led the data department at ConvoyBefore founding Gable.ai, Sanderson and his co-founders, Adrian Kreuziger and Daniel Dicker, led the data department at Convoy, the $4 billion digital freight network that moves thousands of trucks every day across the country via an optimized and connected network. carrier network. Complex data was coming in fast and furious, regarding shipments, shippers, facilities, carriers, trucks, contracts and prices.
Although the company had a modern data stack, using the latest and greatest technologies, no one had confidence in the data: there were constant data quality issues, outages for valuable models and billions of rows of data could not be used. .
"When our data science team and analytics team were trying to understand even simple questions like 'How many shipments have we completed in the last 30 days?', all that complexity made it almost impossible to answer this question." » said Sanderson. “And it was the same problem with machine learning: the models were very, very sensitive and the data scientist had to figure out exactly what data from this very complex system needed to be fed into that model. When data quality was bad, when something suddenly changed, all these sensitive models started to break down and all the predictions they made turned out to be wrong. »
Ultimately, he explained, the problem was a lack of communication between software engineers and ML developers. “Once we helped close this gap, we saw an exponential improvement in data quality almost immediately,” he said.
In order to scale AI, it is essential to resolve communication issues related to data changes, Sanderson emphasized.
"If you don't have a change management system...
What's Your Reaction?
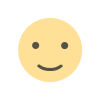
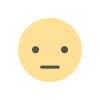
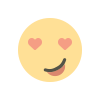
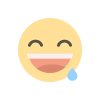
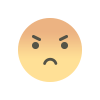
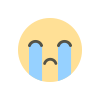
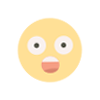