The Secret to Enterprise AI Success: Make It Understandable and Trustworthy
Access our on-demand library to view VB Transform 2023 sessions. Sign up here
The promise of artificial intelligence is finally coming to life. From healthcare to fintech, companies across all industries are rushing to implement LLMs and other forms of machine learning systems to complement their workflows and save time for other more urgent or high-value tasks. But it's all happening so fast that many may be unaware of a key question: how do we know that decision-making machines aren't leaning toward hallucinations?
In healthcare, for example, AI has the potential to predict clinical outcomes or discover drugs. If a model deviates from the correct path in such scenarios, it could provide results that could end up harming a person or worse. No one would want that.
This is where the concept of AI interpretability comes in. It is the process of understanding the reasoning behind decisions or predictions made by machine learning systems and making this information understandable to decision makers and other affected parties with the autonomy to make changes. .
When done right, it can help teams catch unexpected behavior, allowing them to get rid of issues before they cause real damage.
EventVB Transform 2023 on demand
Did you miss a session of VB Transform 2023? Sign up to access the on-demand library for all of our featured sessions.
Register nowBut it's far from child's play.
First, let's understand why AI interpretability is a mustAs critical sectors like healthcare continue to deploy models with minimal human oversight, interpretability of AI has become important to ensure transparency and accountability of the system used.
Transparency ensures that human operators can understand the underlying logic of the ML system and audit it for bias, accuracy, fairness, and adherence to ethical guidelines. In the meantime, accountability ensures that identified shortcomings are addressed on time. The latter is particularly critical in high-stakes areas such as automated credit scoring, medical diagnostics, and autonomous driving, where an AI's decision can have far-reaching consequences.
Beyond that, interpretability of AI also helps build trust and acceptance of AI systems. Essentially, when individuals can understand and validate the reasoning behind decisions made by machines, they are more likely to trust their predictions and responses, resulting in widespread acceptance and adoption. More importantly, when explanations are available, it is easier to answer questions of ethical and legal compliance, whether it concerns discrimination or the use of data.
Interpretability of AI is not an easy taskWhile there are clear benefits to the interpretability of AI, the complexity and opacity of modern machine learning models make it quite a challenge.
Most high-end AI applications today use

Access our on-demand library to view VB Transform 2023 sessions. Sign up here
The promise of artificial intelligence is finally coming to life. From healthcare to fintech, companies across all industries are rushing to implement LLMs and other forms of machine learning systems to complement their workflows and save time for other more urgent or high-value tasks. But it's all happening so fast that many may be unaware of a key question: how do we know that decision-making machines aren't leaning toward hallucinations?
In healthcare, for example, AI has the potential to predict clinical outcomes or discover drugs. If a model deviates from the correct path in such scenarios, it could provide results that could end up harming a person or worse. No one would want that.
This is where the concept of AI interpretability comes in. It is the process of understanding the reasoning behind decisions or predictions made by machine learning systems and making this information understandable to decision makers and other affected parties with the autonomy to make changes. .
When done right, it can help teams catch unexpected behavior, allowing them to get rid of issues before they cause real damage.
EventVB Transform 2023 on demand
Did you miss a session of VB Transform 2023? Sign up to access the on-demand library for all of our featured sessions.
Register nowBut it's far from child's play.
First, let's understand why AI interpretability is a mustAs critical sectors like healthcare continue to deploy models with minimal human oversight, interpretability of AI has become important to ensure transparency and accountability of the system used.
Transparency ensures that human operators can understand the underlying logic of the ML system and audit it for bias, accuracy, fairness, and adherence to ethical guidelines. In the meantime, accountability ensures that identified shortcomings are addressed on time. The latter is particularly critical in high-stakes areas such as automated credit scoring, medical diagnostics, and autonomous driving, where an AI's decision can have far-reaching consequences.
Beyond that, interpretability of AI also helps build trust and acceptance of AI systems. Essentially, when individuals can understand and validate the reasoning behind decisions made by machines, they are more likely to trust their predictions and responses, resulting in widespread acceptance and adoption. More importantly, when explanations are available, it is easier to answer questions of ethical and legal compliance, whether it concerns discrimination or the use of data.
Interpretability of AI is not an easy taskWhile there are clear benefits to the interpretability of AI, the complexity and opacity of modern machine learning models make it quite a challenge.
Most high-end AI applications today use
What's Your Reaction?
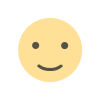
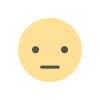
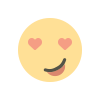
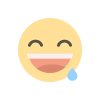
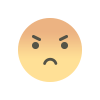
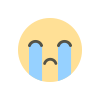
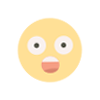