Vector database is a new type of database for the age of AI
Check out all the Smart Security Summit on-demand sessions here.
Businesses across all industries increasingly understand that data-driven decision-making is a necessity to be competitive now, in the next five years, in the next 20 years and beyond. The growth of data – particularly the growth of unstructured data – is off the charts, and recent market research estimates that the global data-driven artificial intelligence (AI) market “will grow at a compound annual growth (CAGR) of 39.4% to reach $422.37 billion by 2028. There is no turning back from the flood of data and the age of AI that is at our doorstep.
Implicit in this reality is that AI can sort and process the flood of data in meaningful ways, not just for tech giants like Alphabet, Meta, and Microsoft with their massive R&D operations and analytics tools. Custom AIs, but for the medium enterprise and even SMBs.
Well-designed AI-based applications sift through extremely large data sets very quickly to generate new insights and ultimately fuel new revenue streams, creating real business value . But nothing of data growth is truly operationalized and democratized without the newcomer: vector databases. These mark a new category of database management and a paradigm shift for utilizing the exponential volumes of untapped unstructured data in object stores. Vector databases offer a bewildering new level of capability for searching unstructured data in particular, but can also handle semi-structured and even structured data.
Unstructured data, such as images, video, audio, and user behaviors, typically don't fit the relational database model; it cannot be easily sorted into row and column relationships. Extremely time-consuming and haphazard methods of managing unstructured data often boil down to manual data markup (think tags and keywords on video platforms).
EventOn-Demand Smart Security Summit
Learn about the essential role of AI and ML in cybersecurity and industry-specific case studies. Watch the on-demand sessions today.
look hereTags can be full of not-so-obvious classifications and relationships. Manual tagging lends itself to traditional lexical searching that matches words and strings exactly. But a semantic search that understands the meaning and context of an image or other unstructured piece of data, along with a search query, is nearly impossible with manual processes.
Enter built-in vectors, also called built-in vectors, feature vectors, or simply built-in. They are numeric values - kinds of coordinates - representing objects or characteristics of unstructured data, such as a component of a photograph, part of a person's shopping profile, selected images in a video, geospatial data or anything that doesn't fit neatly into a relational database table. These integrations enable scalable "similarity search" in a fraction of a second. This means searching for similar items based on closest matches.
Quality data — and insightsIncorporations essentially arise as a computational by-product of an AI model, or more specifically, a machine or deep learning model trained on very large sets of quality input data. To split the import...

Check out all the Smart Security Summit on-demand sessions here.
Businesses across all industries increasingly understand that data-driven decision-making is a necessity to be competitive now, in the next five years, in the next 20 years and beyond. The growth of data – particularly the growth of unstructured data – is off the charts, and recent market research estimates that the global data-driven artificial intelligence (AI) market “will grow at a compound annual growth (CAGR) of 39.4% to reach $422.37 billion by 2028. There is no turning back from the flood of data and the age of AI that is at our doorstep.
Implicit in this reality is that AI can sort and process the flood of data in meaningful ways, not just for tech giants like Alphabet, Meta, and Microsoft with their massive R&D operations and analytics tools. Custom AIs, but for the medium enterprise and even SMBs.
Well-designed AI-based applications sift through extremely large data sets very quickly to generate new insights and ultimately fuel new revenue streams, creating real business value . But nothing of data growth is truly operationalized and democratized without the newcomer: vector databases. These mark a new category of database management and a paradigm shift for utilizing the exponential volumes of untapped unstructured data in object stores. Vector databases offer a bewildering new level of capability for searching unstructured data in particular, but can also handle semi-structured and even structured data.
Unstructured data, such as images, video, audio, and user behaviors, typically don't fit the relational database model; it cannot be easily sorted into row and column relationships. Extremely time-consuming and haphazard methods of managing unstructured data often boil down to manual data markup (think tags and keywords on video platforms).
EventOn-Demand Smart Security Summit
Learn about the essential role of AI and ML in cybersecurity and industry-specific case studies. Watch the on-demand sessions today.
look hereTags can be full of not-so-obvious classifications and relationships. Manual tagging lends itself to traditional lexical searching that matches words and strings exactly. But a semantic search that understands the meaning and context of an image or other unstructured piece of data, along with a search query, is nearly impossible with manual processes.
Enter built-in vectors, also called built-in vectors, feature vectors, or simply built-in. They are numeric values - kinds of coordinates - representing objects or characteristics of unstructured data, such as a component of a photograph, part of a person's shopping profile, selected images in a video, geospatial data or anything that doesn't fit neatly into a relational database table. These integrations enable scalable "similarity search" in a fraction of a second. This means searching for similar items based on closest matches.
Quality data — and insightsIncorporations essentially arise as a computational by-product of an AI model, or more specifically, a machine or deep learning model trained on very large sets of quality input data. To split the import...
What's Your Reaction?
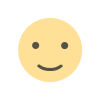
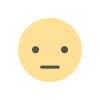
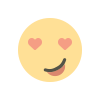
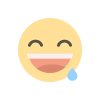
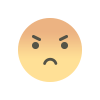
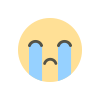
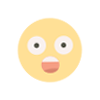