Sleep quality tracking with built-in ML and the Arduino Nano 33 BLE Sense
Sleep quality tracking with built-in ML and the Arduino Nano 33 BLE Sense
Arduino Team — January 10, 2023

Ensuring adequate, restful sleep is essential to maintaining good health, as decreased sleep quality has been shown to cause a host of problems. University of Toronto researchers Zongyan Yao and Xilin Liu recognized this vital aspect of well-being and wanted to create an inexpensive device that could classify the different stages of sleep and report the resulting data wirelessly. p>
Clinical sleep assessment is done by taking polysomnograms, which are basically measurements of the brain, heart, airways, and other physiological characteristics. Zongyan and Xilin decided to use an Arduino Nano 33 BLE Sense to classify single-channel EEG values with a lightweight machine learning model due to its relatively fast processor and available RAM. The training data for the model comes from the extensive Sleep-EDF database which contains several hours of sleep telemetry, including EEG, EOG, airflow and body temperature, although only EEG data has been preserved.

After pre-processing values from the dataset between 0.1 and 10, the team trained a custom 1D convolution model to classify each stage of sleep from wakefulness to eye movement fast (REM), giving an accuracy of 77%. They were able to further increase this metric by performing subject-specific training, further increasing it. In the future, Zongyan and Xilin plan to expand their project further by adding an EEG sensor to enable a wide range of sleep research. More information can be found here in their article.
Image credit: Z. Yao et al.
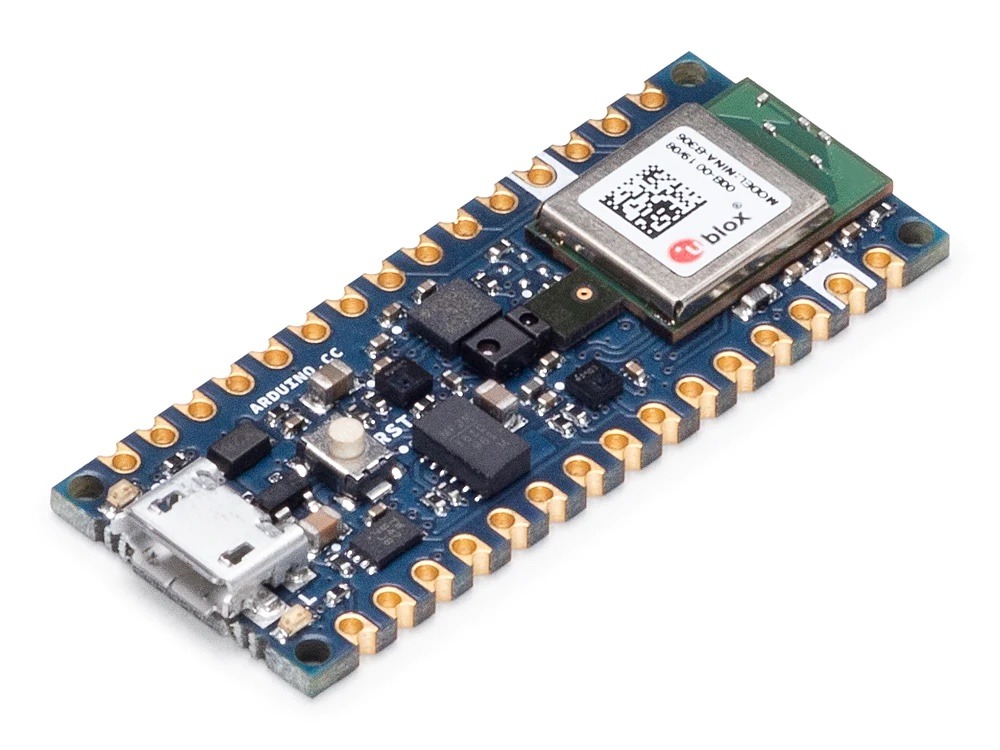
Arduino Team — January 10, 2023

Ensuring adequate, restful sleep is essential to maintaining good health, as decreased sleep quality has been shown to cause a host of problems. University of Toronto researchers Zongyan Yao and Xilin Liu recognized this vital aspect of well-being and wanted to create an inexpensive device that could classify the different stages of sleep and report the resulting data wirelessly. p>
Clinical sleep assessment is done by taking polysomnograms, which are basically measurements of the brain, heart, airways, and other physiological characteristics. Zongyan and Xilin decided to use an Arduino Nano 33 BLE Sense to classify single-channel EEG values with a lightweight machine learning model due to its relatively fast processor and available RAM. The training data for the model comes from the extensive Sleep-EDF database which contains several hours of sleep telemetry, including EEG, EOG, airflow and body temperature, although only EEG data has been preserved.

After pre-processing values from the dataset between 0.1 and 10, the team trained a custom 1D convolution model to classify each stage of sleep from wakefulness to eye movement fast (REM), giving an accuracy of 77%. They were able to further increase this metric by performing subject-specific training, further increasing it. In the future, Zongyan and Xilin plan to expand their project further by adding an EEG sensor to enable a wide range of sleep research. More information can be found here in their article.
Image credit: Z. Yao et al.
What's Your Reaction?
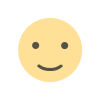
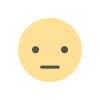
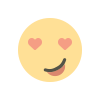
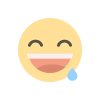
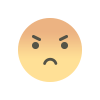
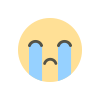
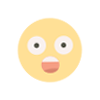